When Causal Inference meets Statistical Analysis
National Conservatory of Arts and Crafts, Paris, France
From April 17th to April 21st
Location: 292 Rue Saint-Martin, 75003 Paris, France

The Colloquium on When Causal Inference meets Statistical Analysis aims to encourage and highlight novel approaches to handle the challenges on causal inferences, causal discovery, causal analysis of machine learning. The colloquium will explore topics related to, but not limited to:
- Causal discovery
- Causal learning and control problems
- Theoretical foundation of causal inference
- Causal inference and active learning
- Causal learning in low data regime
- Reinforcement learning
- Causal machine learning
- Causal generative models
- Benchmark for causal discovery and causal reasoning
Call for Contributions
Full Colloquium Papers
Submission: We invite authors to submit papers of any length between 2 and 6 pages, excluding references. Submissions are not anonymous and must be in English, in PDF format, following the ACM two-column format. Suitable LaTeX and Word templates are available from the ACM website: https://www.acm.org/publications/proceedings-template. Authors should submit their papers via EasyChair https://easychair.org/conferences/?conf=causalstats23.
Note that authors are encouraged to adhere to the best practices of Reproducible Research (RR) by making available data and software tools for reproducing the results reported in their papers. For the sake of persistence and proper authorship attribution, we require using standard repository hosting services such as dataverse, mldata, openml, etc., for data sets, and mloss, Bitbucket, GitHub, etc., for source code. If accepted, authors submitting their work to the colloquium commit themselves to present their paper at the symposium.
Registration
The When Causal Inference meets Statistical Analysis will be held at the National Conservatory of Arts and Crafts, Paris, France from April 17-21, 2023.
Participation is free of charge, but registration is mandatory.
Please register here before April 14th AoE. Please let us know if you need letters for visa application.
All the talks will be at the Auditorium Jean-Baptiste-Say. It is located on the first underground floor and has independent access through entrance number one. See how to get to it here.
Schedule
You can download the program here
Monday, April 17th | |
---|---|
9:00 AM - 9:30 AM | Arrival & welcome coffee |
9:30 AM - 10:00 AM | Welcome & Opening remarks |
10:00 AM - 11:00 AM | Keynote by Bin Yu - Veridical data science with a case study to seek genetic drivers of a heart disease |
11:00 AM - 11:30 AM | Coffee break |
11:30 AM - 12:30 PM |
Oral presentations
|
12:30 PM - 2:00 PM | Lunch (buffet served on the conference premises) |
2:00 PM - 3:00 PM | Keynote by Eric Gaussier - Causal discovery and inference in time series [Slides] |
3:00 PM - 3:30 PM | Coffee break |
3:30 PM - 4:00 PM | Discussion group |
4:00 PM - 6:00 PM |
Oral presentations
|
5:30 PM - 6:00 PM | Discussion group |
6:00 PM - 7:30 PM | Conference reception |
Tuesday, 18 April | |
9:00 AM - 9:30 AM | Arrival & welcome coffee |
9:30 AM - 10:30 AM | Keynote by Yingzhen Li - Towards Causal Deep Generative Models for Sequential Data [Slides] |
10:30 AM - 11:00 AM | Coffee break |
11:00 AM - 11:30 AM | Discussion group |
11:30 AM - 12:30 AM |
Oral presentations
|
12:30 PM - 2:00 PM | Lunch (buffet served on the conference premises) |
2:00 PM - 3:00 PM | Keynote by Daniel Malinsky - |
3:00 PM - 3:30 PM | Coffee break |
3:30 PM - 4:00 PM | Discussion group |
4:00 PM - 6:00 PM |
Oral presentations
|
Wednesday, April 19th | |
9:00 AM - 9:30 AM | Arrival & welcome coffee |
9:30 AM - 10:30 AM | Keynote by Dominik Janzing [Slides] |
10:30 AM - 11:00 AM | Coffee break |
11:00 AM - 11:30 AM | Discussion group |
11:30 AM - 12:30 AM |
Oral presentations
|
12:30 PM - 2:00 PM | Lunch (buffet served on the conference premises) |
2:00 PM - 3:00 PM | Keynote by Chandler Squires [Slides] |
3:00 PM - 4:00 PM | Poster session I |
4:30 PM - 6:00 PM | Social event: Visit The Arts et Métiers Museum |
Thursday, April 20th | |
9:00 AM - 9:30 AM | Arrival & welcome coffee |
9:30 AM - 10:30 AM | Keynote by Jonas Peters |
10:30 AM - 11:00 AM | Coffee break |
11:00 AM - 11:30 AM | Discussion group |
11:30 AM - 12:30 AM |
Oral presentations:
|
12:30 PM - 2:00 PM | Lunch (buffet served on the conference premises) |
2:00 PM - 3:00 PM | Keynote by Jason Hartford |
3:00 PM - 3:30 PM | Oral presentation:
|
3:30 PM - 4:30 PM | Keynote by Krikamol Muandet |
4:30 PM - 6:30 PM | Poster session II |
7:30 PM - 10:30 PM | Conference dinner at Le Procope restaurant |
Friday, April 21st | |
9:00 AM - 9:30 AM | Arrival & welcome coffee |
9:30 AM - 10:30 AM | Keynote by Antoine Chambaz [Slides] |
10:30 AM - 11:00 AM | Coffee break |
11:00 AM - 11:30 AM | Discussion group |
11:30 AM - 12:30 AM | Oral presentations: |
12:30 PM - 2:00 PM | Lunch (buffet served on the conference premises) |
2:00 PM - 3:00 PM | Keynote by Elina Robeva [Slides] |
3:00 PM - 4:30 PM |
Oral presentations:
|
4:30 PM - 5:30 PM | Wrap-up & cocktail |
Keynote Speakers
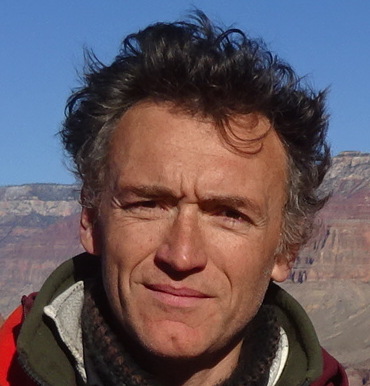
Paris Cité University
Title: Learning, evaluating and analyzing a recommendation rule for early blood transfer in the ICU
Abstract
Severely injured patients experiencing hemorrhagic shock often require massive transfusion. Early transfusion of blood products (plasma, platelets and red blood cells) is common and associated with improved outcomes in the hospital. However, determining a right amount of blood products is still a matter of scientific debate. The speaker will present and discuss a methodology to learn, evaluate and analyse a recommendation rule for early blood transfer in the ICU. The study uses data from the French Traumabase, a French observatory for Major Trauma. This is a joint work with Pan Zhao (Inria), Julie Josse (Inria), Nicolas Gatulle (APHP) and the Traumabase Group.
Antoine Chambaz is a professor at Université Paris Cité, member of the applied mathematics laboratory (MAP5). He is the head of the Statistics group since June 2018, and the director of the FP2M research federation. From 2012 to 2017, he was a member of Modal'X, the stochastic modeling laboratory of Paris Nanterre University. He chaired Modal'X from February 2014 to October 2017. His main research interest is in theoretical, computational and applied statistics.
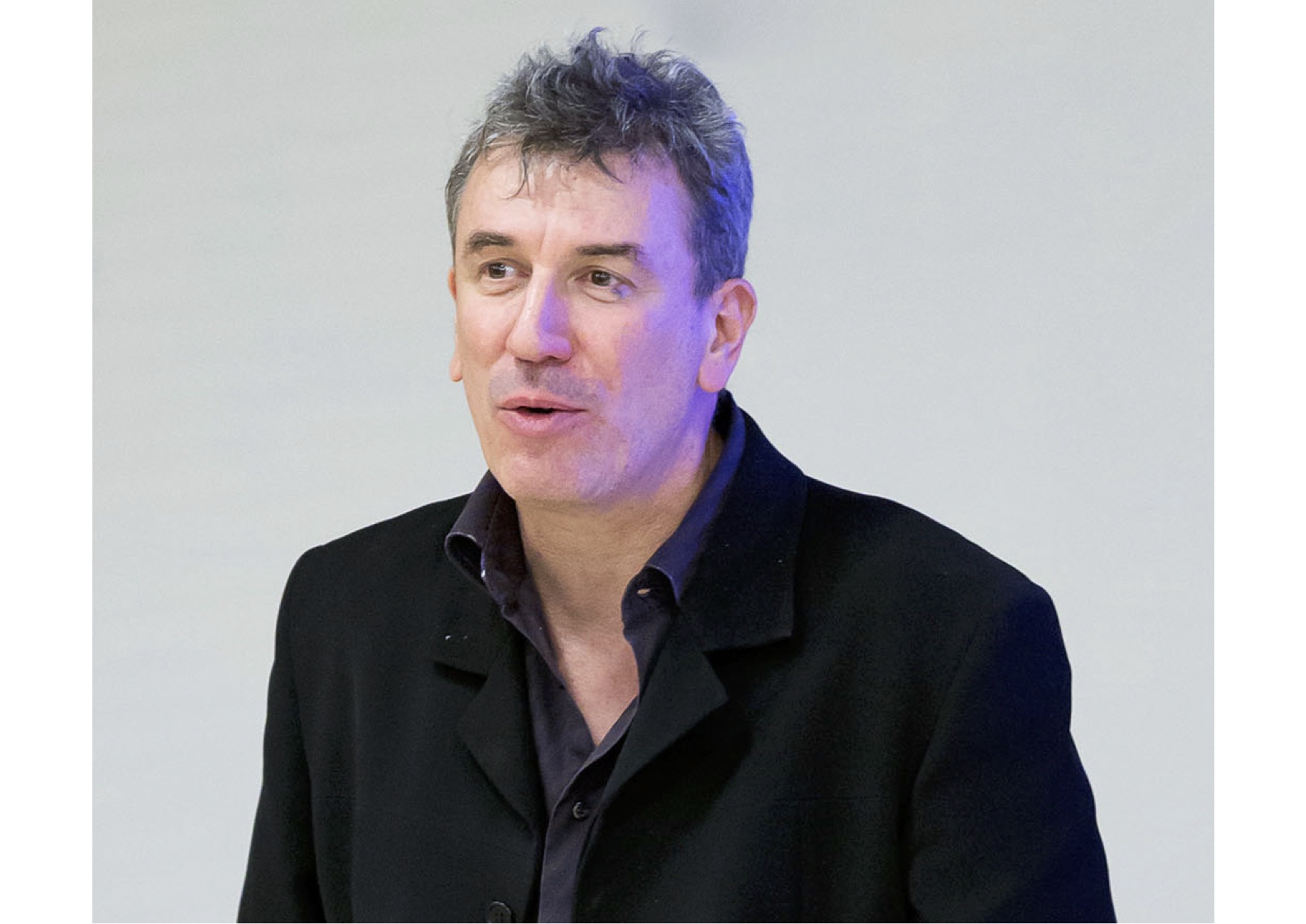
University of Grenoble Alpes
Title: Causal discovery and inference in time series
Abstract
Time series are present in various forms in many different domains, as healthcare, Industry 4.0, monitoring systems or energy management to name but a few. Despite the presence of temporal information, discovering causal relations and identifying interventions in time series are not easy tasks, in particular when one is dealing with "incomplete" graphs. We will first discuss which causal graphs are relevant to time series and present methods to extract them from observational data and identify interventions in them.
Eric Gaussier is a professor of Computer Science at University Grenoble Alps and Director of the Grenoble Interdisciplinary Institute in Artificial Intelligence. His research lies at the intersection machine learning, information retrieval and computational linguistics. The data on which he has primarily worked are large-scale, multi-lingual collections and he is particularly interested in theoretical models that explain and take into account the properties of the collections considered, and in large-scale, practical implementations of these models. He has also been interested in modeling how (textual) information is shared in social (content) networks, and how such networks evolve over time. He has also worked on improving job scheduling techniques through machine learning, and in learning representations for different types of sequences, as texts and time series.

Recursion Pharmaceutical
Title: Toward causal inference from high-dimensional observations
Abstract
Over the last decade, biologists have developed a multitude of tools with which we can generate high-dimensional observations of biological systems. These tools either directly measure causal variables (e.g. the expression levels of genes) or they measure unstructured proxies for the causal variables (e.g. the pixels in an image of a cell). When these high-dimensional causal variables are directly measured, the relationship with outcomes of interest typically remains confounded so we need to rely on experimentation to identify causal effects. The challenge is (1) we often cannot intervene directly on the causal variables, and instead perturb them indirectly, and (2) the number of experiments that we can practically run is typically far fewer than the number of causal variables of interest. I will discuss how we can use instrumental variable methods to estimate causal effects in this regime, and show when we can estimate the causal effect with relatively few experiments despite being underspecified. The second part of my talk will focus on representation learning which is needed when we measure unstructured proxies for the causal variables. These modalities, such as images or sensor data can often be collected cheaply in experiments, but they are challenging to use in a causal inference pipeline without extensive feature engineering or labelling to extract underlying latent factors. I will present results from a series of recent papers that describe when we can disentangle latent variables with identifiability guarantees.
Jason Hartford is currently a Senior Research Scientist at Recursion Pharmaceutical. Previously, was a postdoc with Prof Yoshua Bengio at Mila where he worked on causal representation learning. Before joining Mila, he completed his Master's and PhD at the University of British Columbia with Prof Kevin Leyton-Brown where he worked on deep learning-based estimators of causal effects, and enforcing symmetries in neural networks. During his PhD he had internships at Microsoft Research New England and Redmond where he worked on deep learning approaches for causal inference.

Amazon Research
Title: Causal insights from merging data sets and merging data sets via causal insights
Abstract
While humans often draw causal conclusions from putting observations into the broader context of causal knowledge, AI still needs to develop these techniques. I show how causal insights can be obtained from the synergy of datasets referring to different sets of variables and argue that causal hypotheses then predict joint properties of variables that have never been observed together. This way, causal discovery becomes a prediction task in which additional variable sets play the role of additional data points in traditional iid learning. For instance, a causal DAG can be seen as a binary classifier that tells us which conditional independences are valid, which then enables a statistical learning theory for learning DAGs.
I describe "Causal MaxEnt" (a modified version of MaxEnt that is asymmetric with respect to causal directions) as one potential approach to infer DAGs and properties of the joint distribution from a set of marginal distributions of subsets of variables and derive causal conclusions for toy examples.
Further reading:
- [1] Dominik Janzing: Merging joint distributions via causal model classes with low VC dimension, arxiv:1804.03206
- [2] Sergio Garrido Mejia, Elke Kirschbaum, Dominik Janzing: Obtaining causal information by merging data sets with MaxEnt. AISTATS 2022
- [3] Dominik Janzing: Causal versions of Maximum Entropy and Principle of Insufficient Reason, Journal of Causal Inference 2021.
Dominik Janzing is a Principal Research Scientist at Amazon Research. Since 2003, he works on causal inference from statistical data and the foundation of new causal inference rules. From 1995 to 2007, he worked on quantum information theory, quantum computing, complexity theory, and thermodynamics. This work can be summarized as physics of Information and he thinks that causal inference also relies on assumptions that connect physics with information. He believes that the science of causality is "abstract physics".

Imperial College London
Title: Towards Causal Deep Generative Models for Sequential Data
Abstract
One of my research dreams is to build a high-resolution video generation model that enables granularity controls in e.g., the scene appearance and the interactions between objects. I tried, and then realised the need of me inventing deep learning tricks for this goal is due to the issue of non-identifiability in my sequential deep generative models. In this talk I will discuss our research towards developing identifiable deep generative models in sequence modelling, and share some recent and on-going works regarding switching dynamic models. Throughout the talk I will highlight the balance between causality "Theorist" and deep learning "Alchemist", and discuss my opinions on the future of causal deep generative modelling research.
Yingzhen Li is an assistant professor at the Department of Computing at Imperial College London. She is interested in building reliable machine learning systems which can generalize to unseen environments. She approaches this goal by using probabilistic modeling and representation learning, some of her research topics include: (deep) probabilistic graphical model design, fast and accurate (Bayesian) inference/computation techniques, uncertainty quantification for computation and downstream tasks, and robust and adaptive machine learning systems.

Columbia University
Title: A Cautious Approach To Constraint-Based Causal Model Selection Based on Equivalence Tests
Abstract
Causal graphical models are used many scientific domains to represent important causal assumptions about the processes that underlie collected data. The focus of this work is on graphical structure learning (a.k.a. causal discovery or model selection) for the “downstream” purpose of using the estimated graph for subsequent causal inference tasks, such as establishing the identifying formula for some causal effect of interest and then estimating it. An obstacle to having confidence in existing procedures in applied health science settings is that they tend to estimate structures that are overly sparse, i.e., missing too many edges. However, statistical “caution” (or “conservativism”) would err on the side of more dense graphs rather than more sparse graphs. This paper proposes to reformulate the conditional independence hypothesis tests of classical constraint-based algorithms as equivalence tests: test the null hypothesis of association greater than some (user-chosen, sample-size dependent) threshold, rather than test the null of no association. We argue this addresses several important statistical issues in applied causal model selection and leads to procedures with desirable behaviors and properties.
Daniel Malinsky is an assistant professor of Biostatistics in the Mailman School of Public Health at Columbia University. His research focuses mostly on causal inference: developing statistical methods and machine learning tools to support inference about treatment effects, interventions, and policies. His current research topics include structure learning (a.k.a. causal discovery or causal model selection), semiparametric inference, time series analysis, and missing data. He also works on algorithmic fairness: understanding and counteracting the biases introduced by data science tools deployed in socially-impactful settings. Finally, He has interests in the philosophy of science and the foundations of statistics.
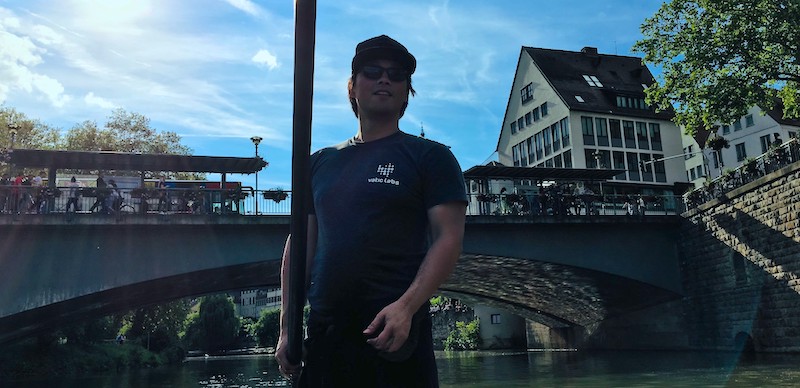
CISPA - Helmholtz Center for Information Security
Title: Reliable Machine Learning with Instruments
Abstract
Society is made up of a set of diverse individuals, demographic groups, and institutions. Learning and deploying algorithmic models across these heterogeneous environments face a set of various trade-offs. In order to develop reliable machine learning algorithms that can interact successfully with the real world, it is necessary to deal with such heterogeneity. In this talk, I will focus on how to employ an instrumental variable (IV) to alleviate the impact of unobserved confounders on the credibility of algorithmic decision-making and the reliability of machine learning models that are learned from observational and heterogeneous data. In particular, I will present how we can leverage tools from machine learning, namely, kernel methods and deep learning, to solve potentially ill-posed non-linear IV regression and proxy variable problems. Lastly, I will argue that a better understanding of the ways in which our data are generated and how our models can influence them will be crucial for reliable human-machine interactions, especially when gaining full information about data may not be possible.
Krikamol Muandet is a chief scientist and tenure-track faculty (fast track) at CISPA - Helmholtz Center for Information Security which is within the Helmholtz Association. From 2018 to 2022, he was a research group leader affiliated with the Empirical Inference Department at Max Planck Institute for Intelligent Systems, Tübingen, Germany. From January 2016 to December 2017, he was a lecturer at the Department of Mathematics, Faculty of Science, Mahidol University in Thailand. He graduated summa cum laude with a PhD degree specializing in kernel methods in machine learning. His PhD advisor was Prof. Bernhard Schölkopf. He also obtained a master's degree with distinction in machine learning from University College London (UCL), United Kingdom. At UCL, He worked primarily in the Gatsby Unit with Prof. Yee Whye Teh. He has a broad interest in machine learning. His current research aims at creating and understanding intelligent machines that can learn via both observation and experimentation.
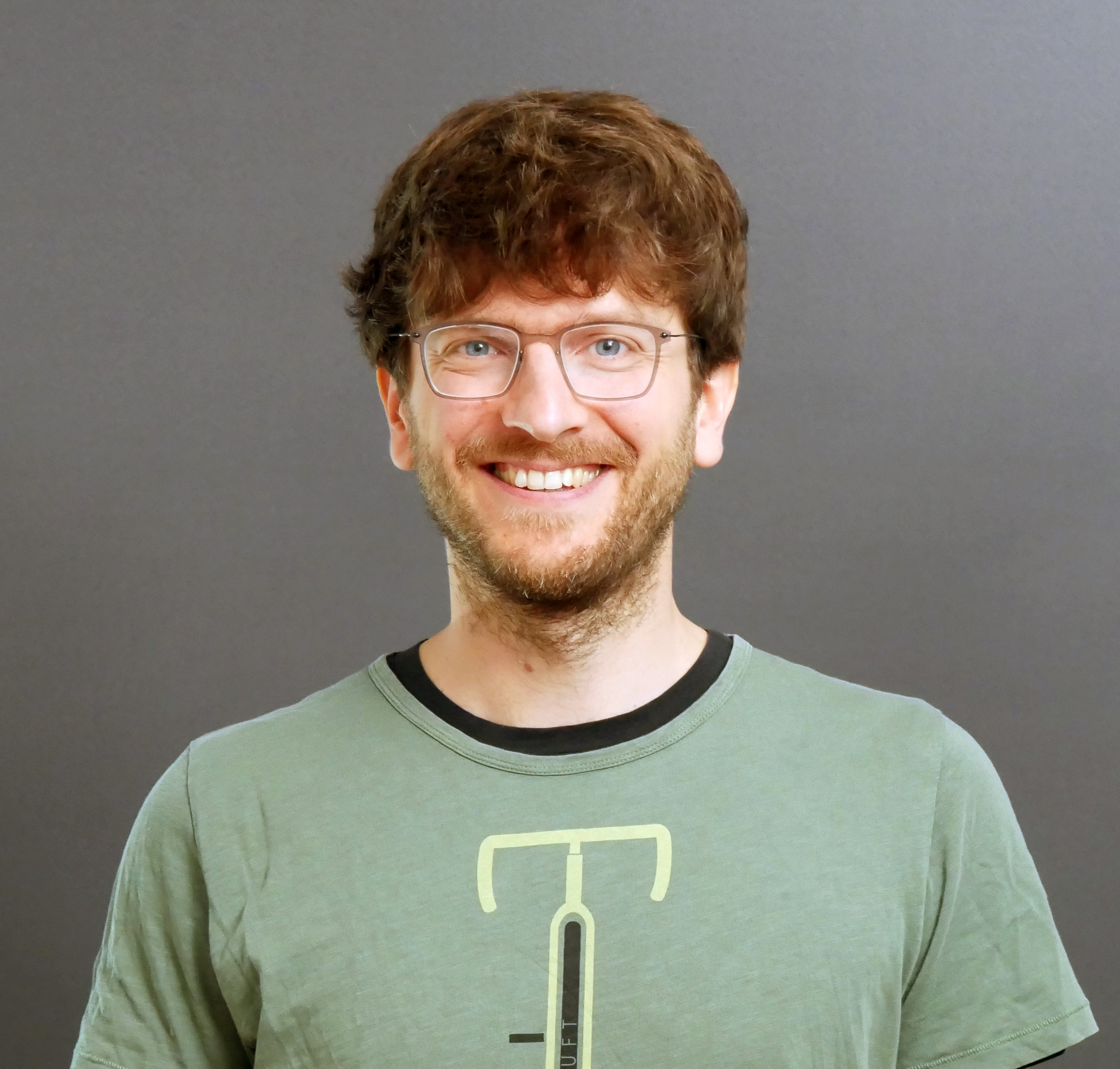
ETH Zurich
Title: Statistical Testing under Distributional Shifts and its Application to Causality
Abstract
We discuss the problem of statistical testing under distributional shifts. In such problems, we are interested in the hypothesis P in H0 for a target distribution P, but observe data from a different distribution Q. We assume that P is related to Q through a known shift tau and formally introduce hypothesis testing in this setting. We propose a general testing procedure that first resamples from the observed data to construct an auxiliary data set and then applies an existing test in the target domain. This proposal comes with theoretical guarantees. Testing under distributional shifts allows us to tackle a diverse set of problems. We argue that it may prove useful in reinforcement learning and covariate shift, we show how it reduces conditional to unconditional independence testing and we provide example applications in causal inference. This is joint work with Nikolaj Thams, Sorawit Saenkyongam, and Niklas Pfister.

University of British Columbia
Title: Learning Linear Non-Gaussian Causal Models via Higher Moment Relationships
Abstract
In this talk we will discuss the problem of learning the directed graph for a linear non-Gaussian causal model. We will specifically address the cases where the graph may have cycles or there might be hidden variables. While ICA methods are able to recover the correct graph, they do not always guarantee to have found the optimal solution. Our methods are based on using specific relationships that hold among the 2nd and 3rd moments of the random vector and can help us characterize the graph. While in the acyclic case, the directed graph can be found uniquely, when cycles are allowed the graph can be learned up to an equivalence class. We give a description of all the graphs that each equivalence class consists of. We then give an algorithm based on relationships among the second and third order moments of the random vector that recovers the equivalence class of the graph, assuming the graph lies in a specific family of cyclic graphs. This is joint work in progress with Mathias Drton, Marina Garrote-Lopez, and Niko Nikov.
Elina Robeva is an assistant professor at the Department of Mathematics in the University of British Columbia in Vancouver, Canada. Her research lies at the intersection of mathematical statistics, machine learning, combinatorics, multi-linear algebra, and applied algebraic geometry. She particularly enjoys discovering mathematical structure which is inherently responsible for the successful solution to a statistical problem. Most recently she has been working on the theory of linear causal models, structured tensor decompositions, shape-constrained density estimation, and super-resolution imaging.
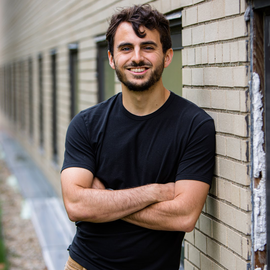
MIT
Title: Beyond ICA: Causal Disentanglement via Interventions
Abstract
Traditional methods for representation learning, such as independent component analysis (ICA), seek representations of observed data in terms of a set of independent variables. However, human reasoning relies on variables which are not statistically independent, such as height and weight. The emerging field of causal representation learning takes the view that the variables in a representation should not be statistically independent, but rather that they should be causally autonomous. From a generative modeling perspective, we may view learning such a representation as an inference problem, which we call "causal disentanglement". In this talk, I will outline a research program which aims to build a computational and statistical theory of causal disentanglement. I will discuss initial results in this program, including our own work proving identifiability of causal representations from interventions in a linear setting. I will conclude with an overview of future applications for these methods in biology, healthcare, and human-AI interaction.
Chandler Squires is PhD student at MIT. His research is centered on learning the effects of intervening in complex systems, with a particular focus on cellular biology and healthcare. This spans causal structure learning, active learning for causal structure discovery, causal representation learning, and treatment effect estimation. He is co-advised by Caroline Uhler and David Sontag.

University of California, Berkeley
Title: Veridical data science with a case study to seek genetic drivers of a heart disease
Abstract
"AI is like nuclear energy–both promising and dangerous." - Bill Gates, 2019. Data Science is a pillar of AI and has driven most of recent cutting-edge discoveries in biomedical research and beyond. Human judgment calls are ubiquitous at every step of a data science life cycle, e.g., in problem formulation, choosing data cleaning methods, predictive algorithms and data perturbations. Such judgment calls are often responsible for the "dangers" of AI. To mitigate these dangers, we introduce in this talk a framework based on three core principles: Predictability, Computability and Stability (PCS). The PCS framework unifies and expands on the ideas and best practices of statistics and machine learning. It emphasizes reality check through predictability and takes a full account of uncertainty sources in the whole data science life cycle including those from human judgment calls such as those in data curation/cleaning. PCS consists of a workflow and documentation and is supported by our software package veridical or v-flow. Moreover, we illustrate the usefulness of PCS in the development of iterative random forests (iRF) for predictable and stable non-linear interaction discovery (in collaboration with the Brown Lab at LBNL and Berkeley Statistics). Finally, in the pursuit of genetic drivers of a heart disease called hypertrophic cardiomyopathy (HCM) as a CZ Biohub project in collaboration with the Ashley Lab at Stanford Medical School and others, we use iRF and UK Biobank data to recommend gene-gene interaction targets for knock-down experiments. We then analyze the experimental data to show promising findings about genetic drivers for HCM.
Bin Yu is Chancellor's Distinguished Professor and Class of 1936 Second Chair in Statistics, EECS, and Computational Biology at UC Berkeley. Her research focuses on statistical machine learning practice and theory and interdisciplinary data problems in neuroscience, genomics, and precision medicine. She and her team developed in context iterative random forests (iRF), hierarchical shrinkage (HS) for decision trees, Fast Interpretable Greedy-Tree Sums (FIGS), stability-driven NMF (staNMF), and adaptive wavelet distillation (AWD) from deep learning models. She is a member of the National Academy of Sciences and American Academy of Arts and Sciences. She was a Guggenheim Fellow. She is to deliver the IMS Wald Lectures and COPSS DAAL (formerly Fisher) Lecture at JSM in Aug. 2023. She holds an Honorary Doctorate from The University of Lausanne.
Oral presentations
Posters
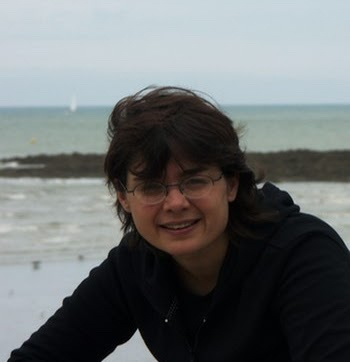
University of Lorraine
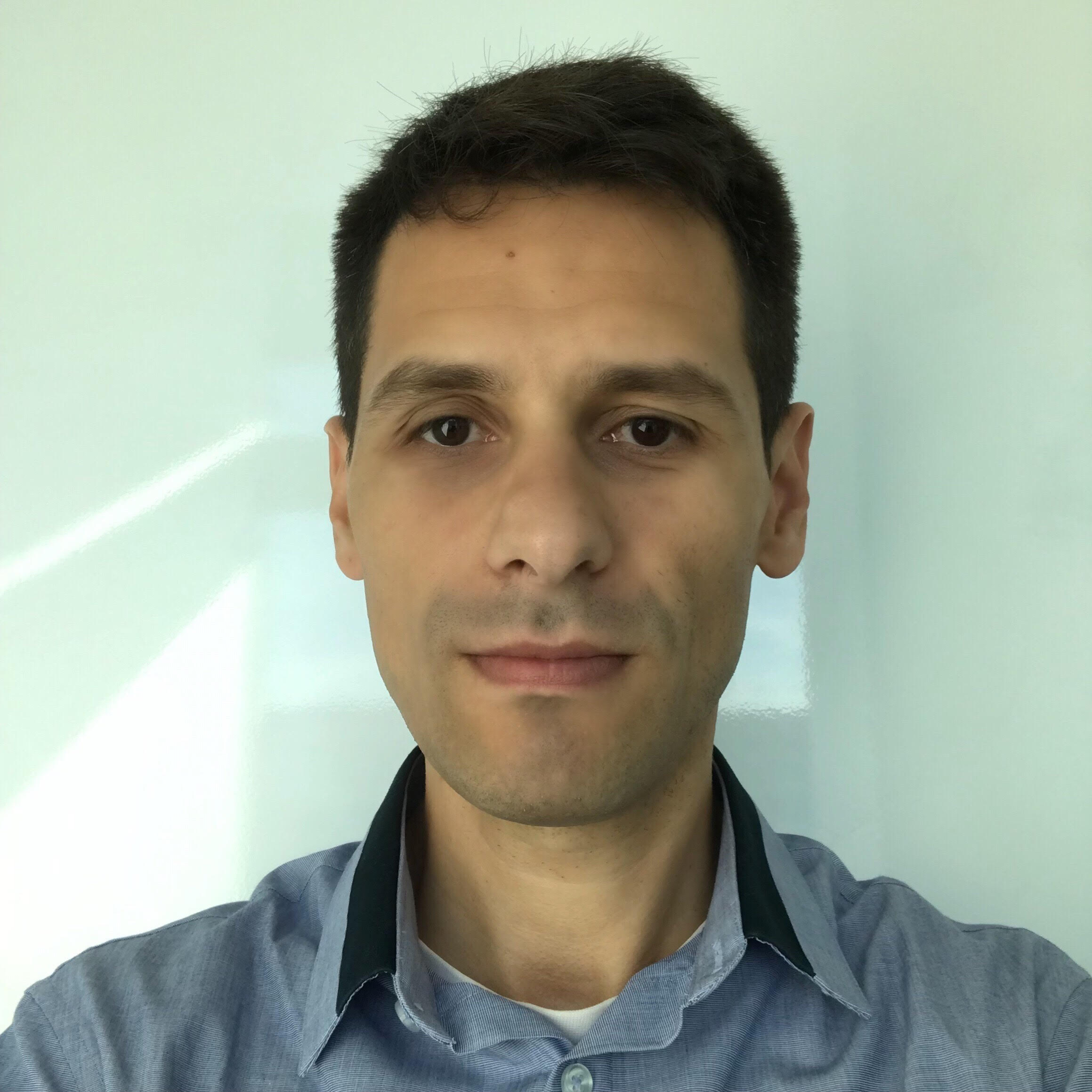
TAU, INRIA, Paris-Saclay University
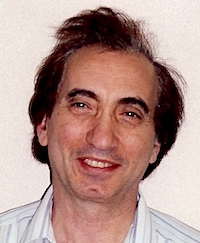
Paris-Saclay University
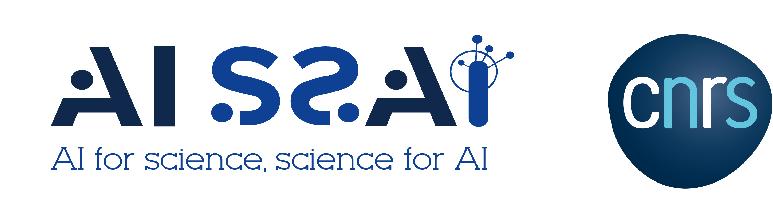
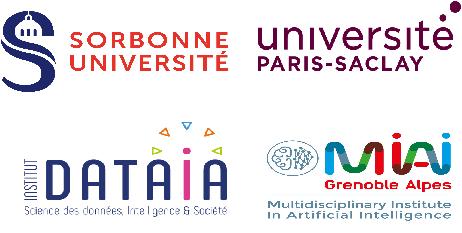
Code of Conduct
Our When Causal Inference meets Statistical Analysis is dedicated to providing a harassment-free experience for everyone, regardless of gender, gender identity and expression, age, sexual orientation, disability, physical appearance, body size, race, ethnicity, religion (or lack thereof), or technology choices. We do not tolerate harassment of participants in any form. Sexual language and imagery is not appropriate for any venue, including talks, workshops, parties, Twitter and other online media. Participants violating these rules may be sanctioned or expelled from the event at the discretion of the conference organizers. If you have any concerns about possible violation of the policies, please contact the organizers (organizers.quarter.causality@gmail.com) as soon as possible.