Causality in Practice
Institute Pascal, Orsay, France
From June 12th to June 16th, 2023
Location: Institute Pascal 530 rue Andre Riviere, Orsay
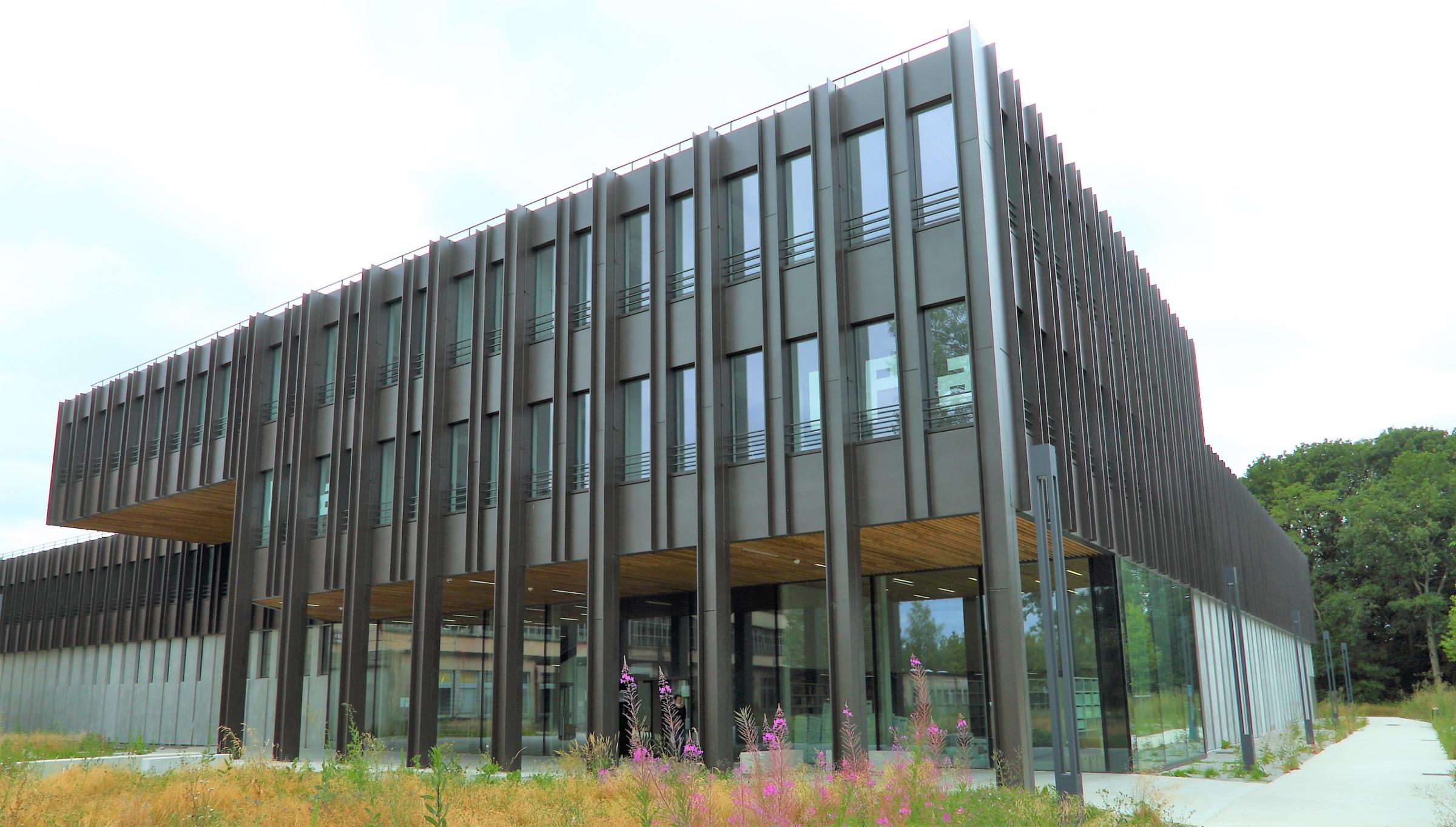
Causal and effect questions are the foundation for numerous scientific disciplines, providing a framework for formulating and comprehending them under diverse conditions. In recent years, there has been a notable interdisciplinary effort to develop machine learning and statistical analysis techniques to address causality-related challenges. Simultaneously, the causal perspective has been used to formulate and understand various machine-learning problems.
The colloquium on Causality in Practice aims to facilitate discussions and knowledge sharing among researchers and practitioners who have applied causality in various domains. This event presents a unique opportunity to explore the practical aspects of causal reasoning. Attendees will have the chance to learn from experts in different fields and discover diverse real-world applications of causality. Moreover, participants can present their work at a poster session.
Registration
The Causality in Practice will be held at the Institute Pascal, Orsay, France from June 12-16, 2023.
Participation is free of charge, but registration is mandatory.
Please register here.
Venue
Institute Pascal is situated on the Orsay campus of the Paris-Saclay University, approximately 25 km south-west of Paris. The campus is accessible from Paris via the RER B and the building can be reached in approximately 50 minutes from the Châtelet-Les Halles station in the center of Paris.
The address is 530 rue Andre Riviere, Orsay (GPS coordinates: 48â—¦42024.200N2â—¦10038.100E)
Social dinner
The social dinner will be at Le Gramophone restaurant. The address is 27 Bd Dubreuil, 91400 Orsay, France.Schedule
Monday | Tuesday | Wednesday | Thursday | Friday | ||
---|---|---|---|---|---|---|
9:00 | Welcome coffee | |||||
9:30 | Ricardo Silva, University College London | Mélanie Prague, Inria | Raphaël Porcher, Université Paris Cité | Qingyuan Zhao, University of Cambridge | Johannes Textor, Data Science, Radboud University Nijmegen, The Netherlands | |
10:30 | Coffee break | |||||
10:45 | Martin Huber, University of Fribourg | Elise Dumas, Institute Curie | Céline Beji, Université Paris Cité | Pedro Sanchez, University of Edinburgh | Audrey Poinsot, Ekimetrics, Inria, Paris-Saclay University | |
11:45 | Jakob Zeitler, University College London | Judith Abécassis, Inria | Erwan Scornet, Ecole Polytechnique, CMAP | Marcel Ribeiro-Dantas, Seqera Labs | Philippe Brouillard, Université de Montréal, Mila | |
12:45 | Lunch break | |||||
14:15 | Benjamin Heymann, Criteo and Michel De Lara, ENPC |
Sander Beckers, University of Amsterdam | François Grolleau, Université Paris Cité | Charles Assaad, EasyVista | ||
15:15 | Coffee break | |||||
15:30 | Limor Gultchin, University of Oxford, The Alan Turing Institute | Matej Zečević, TU Darmstadt | Florie Bouvier, Université Paris Cité | Poster session | ||
16:30 | Miguel Monteiro, Imperial College London, Qureight | Dhanya Sridhar, University of Montreal, Mila | Carlos Cinelli, University of Washington | Ying Jin, Stanford University | ||
17:30 | Cocktail | |||||
19:00 | Social dinner |
Speakers (Alphabetical order)
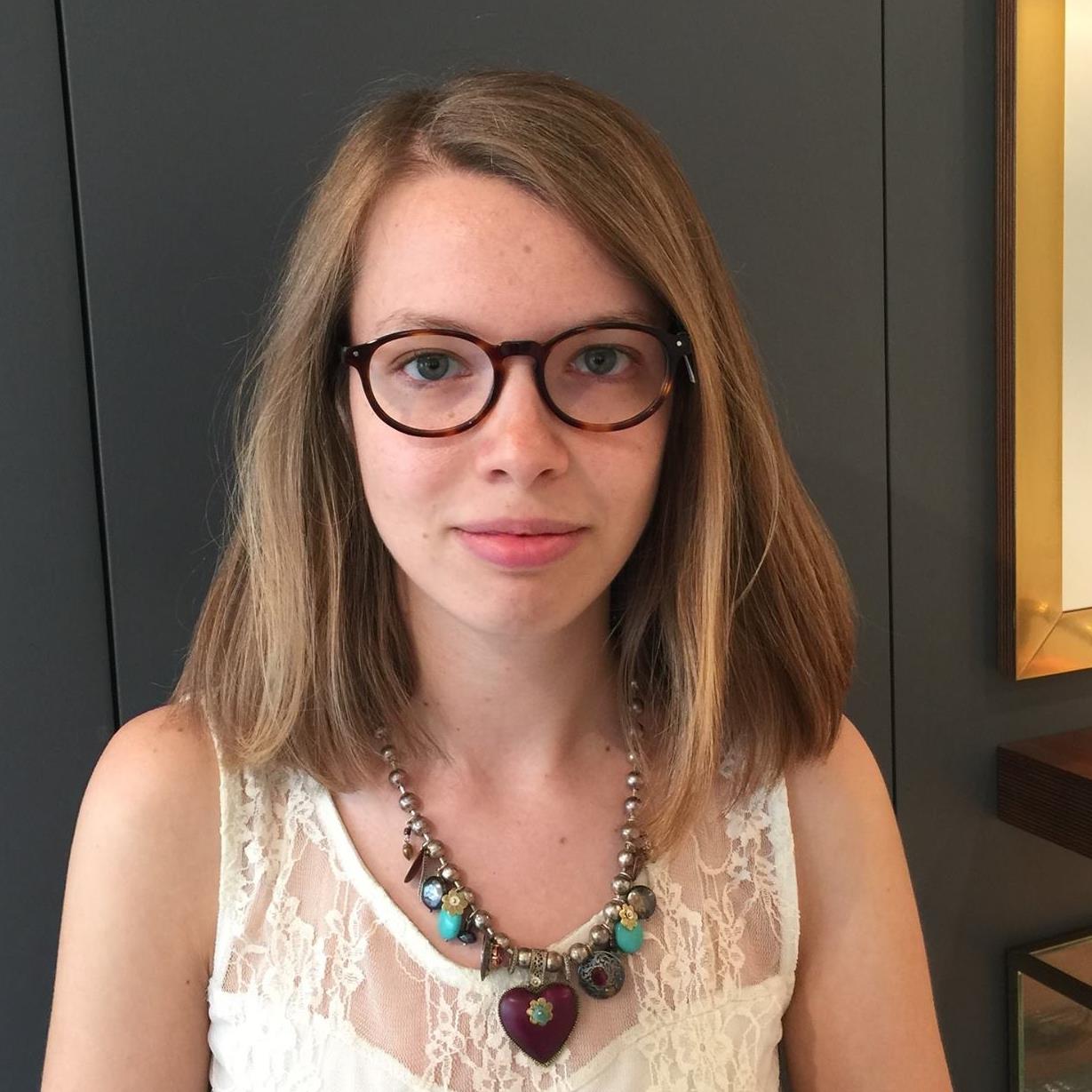
Inria
Title: Exploring cognition in the UK Biobank with causal mediation analysis
Abstract
Causal inference in observational studies is primarily used to measure the causal effect of a treatment on an outcome. Nevertheless, in a lot of fields, disentangling the mechanism of action is just as important, as it allows us to identify potential intermediate intervention targets, and more generally, deepen our understanding of the processes that lead to the observed outcome. Causal mediation analysis aims at separating the (total) causal effect into two components: an indirect effect through a third (group of) variable(s) called mediator(s), and a direct effect without intermediate. Most of existing methods are dedicated to the case of a one-dimension binary mediator, while the problem of considering several mediators is increasingly considered, especially for high dimension settings, such as gene expression or medical imaging. We perform a thorough evaluation of estimators for direct and indirect effects in the context of mediation analysis for binary, continuous and multi-dimensional mediators. We consider both parametric and semi-parametric estimators, and assess the relevance of several implementation variants, in particular regularization, non-parametric models for nuisance parameters estimation, probability calibration and cross-fitting. We then apply the mediation analysis framework to the exploration of cognitive function in a population of around 40,000 UK Biobank participants who underwent brain MRI, and a complete physical, sociodemographic, cognitive, and medical assessment. This prospective cohort is unique by its size, and the opportunity to disentangle the social and physiological components of human cognition. In a preliminary study, we consider several treatments believed to affect cognitive abilities. We found evidence of mediation by the brain structure for several of those exposures, in particular the ones that the organism physiology.
Judith Abécassis is a researcher in the Soda team at Inria Saclay. She works at the intersection between statistical methods in causal inference and medical applications to provide relevant and potentially actionable insights for better patient care. Before that, she was a postdoc in the Parietal team (now MIND) at Inria Saclay under the supervision of Bertrand Thirion and Julie Josse, working on causal mediation analysis with an application to brain imaging in the UK Biobank. She holds a Ph.D. in Bioinformatics at the Center for Computational Biology from the Ecole Mines ParisTech and the RT2 Lab (tumor residue and response to treatment) at Institut Curie under the supervision of Jean-Philippe Vert and Fabien Reyal, where she focused on the analysis of high-throughput sequencing data from cancer genomes.
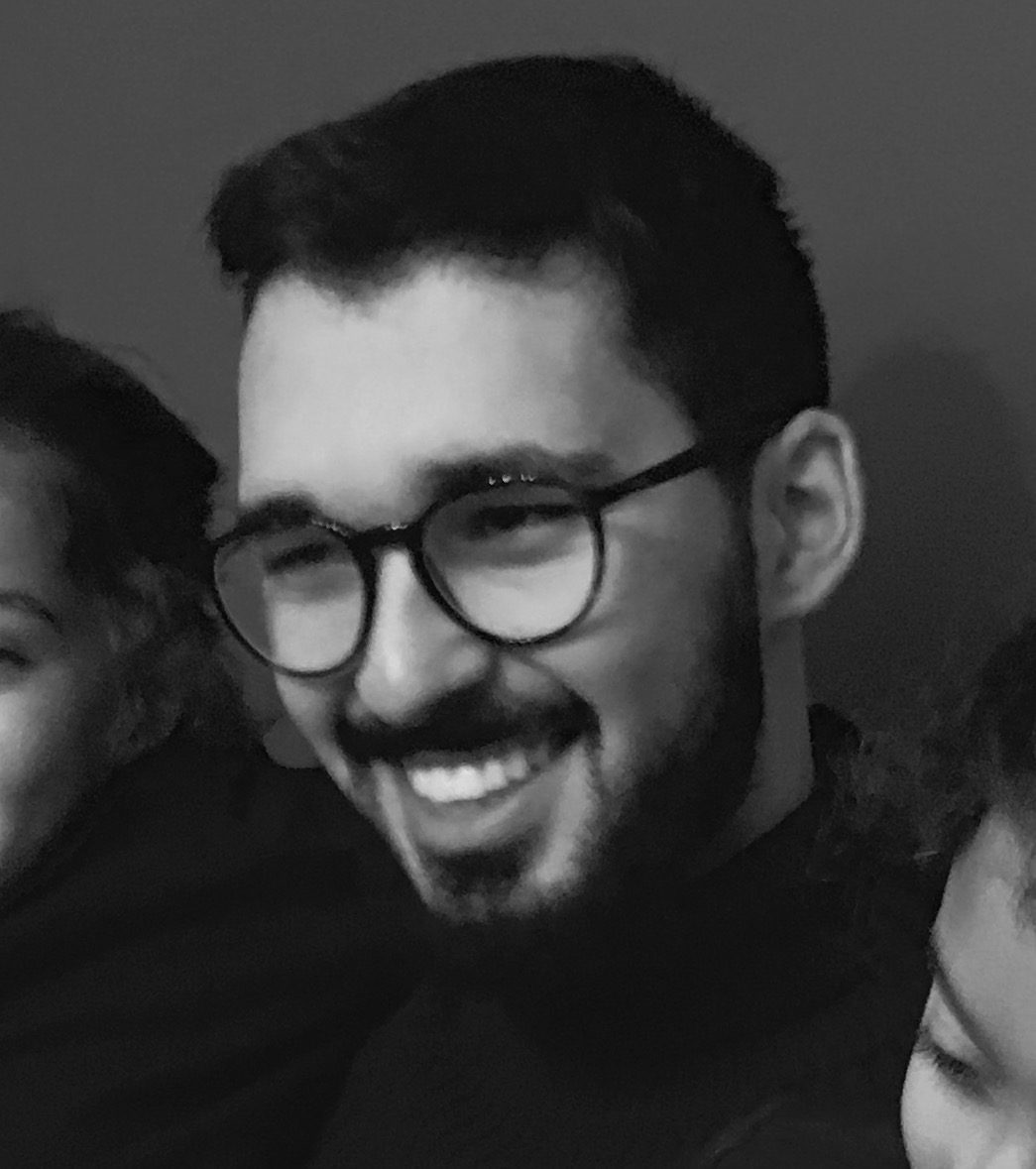
EasyVista
Title: Root cause analysis in IT monitoring systems
Abstract
Automatic root cause identification is a challenging and important task in IT monitoring systems, where failures and anomalies can have severe consequences for businesses and customers. Traditional methods rely on manual rules, heuristics, or statistical correlations to identify the root causes of incidents, but often fail to capture the complex and dynamic dependencies among IT components. In this talk, a new framework will be presented that leverages causal discovery and causal reasoning to automatically infer root causes of IT incidents from observational time series data. The effectiveness of the framework will be demonstrated on simulated data as well as on real world monitoring data.
Charles K. Assaad is a research scientist at EasyVista in the lab Team of EV Observe. He received his Ph.D. from Université Grenoble Alpes (with Emilie Devijver and Eric Gaussier) and his engineering degree from National School of Computer Science for Industry and Business (ENSIIE). His work focuses mainly on causal discovery and causal reasoning, which comprise learning causal structures from purely observational data and studying the reasonings one can do with the inferred causal structures. His research interests include causal discovery, root cause analysis, anomaly detection, time series analysis, causal reasoning, history of causation, machine learning, and information theory.
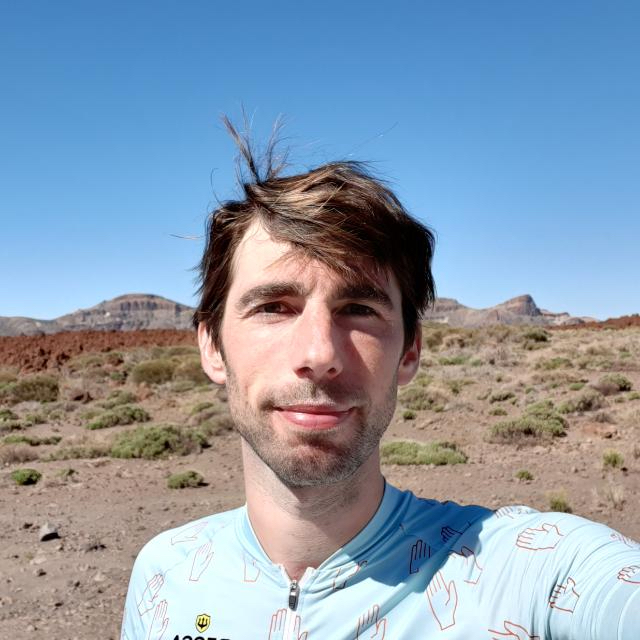
University of Amsterdam
Title: Causal Analysis of Harm
Abstract
As autonomous systems rapidly become ubiquitous, there is a growing need for a legal and regulatory framework that addresses when and how such a system harms someone. There have been several attempts within the philosophy literature to define harm, but none of them has proven capable of dealing with the many examples that have been presented, leading some to suggest that the notion of harm should be abandoned and “replaced by more well-behaved notions”. As harm is generally something that is caused, most of these definitions have involved causality at some level. Yet surprisingly, none of them makes use of causal models and the definitions of actual causality that they can express. In this paper we formally define a qualitative notion of harm that uses causal models and is based on a well-known definition of actual causality. The key features of our definition are that it is based on contrastive causation and uses a default utility to which the utility of actual outcomes is compared. We show that our definition is able to handle the examples from the literature, and illustrate its importance for reasoning about situations involving autonomous systems. The paper is available here. It is a joint work with Joseph Y. Halpern (Cornell University) and Hana Chockler (King's College London)
Sander Beckers is a postdoctoral researcher at the Institute for Logic, Language, and Computation from the University of Amsterdam. He works on a variety of topics involving causation and causal modeling. Examples include the use of causal models to construct and discuss formal definitions of actual causation and their properties; the combination of causal models and the descriptions of actual causation to formally define other essential notions, such as harm, responsibility, and explanation, and the extension of the framework of causal models itself, so that they can express a broader range of relations. He is a philosopher, and his philosophical interests branch out to diverse areas that encompass the philosophy of Wittgenstein, the limitations of scientific knowledge, cultural relativism, and any topic in formal philosophy.
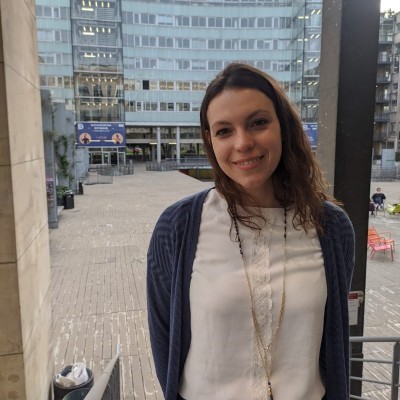
Université Paris Cité
Title: Latent distribution estimation for the evaluation of the complier average causal effect
Abstract
The complier average causal effect (CACE) estimator, defined as the average of potential outcomes in the latent sub-population that complies with their assigned treatment, is more and more used in clinical trials to study the effect of a medication or an intervention rather than the effect of its prescription. Although advanced methods such as instrumental variables and G-estimation have been developed, it requires strong assumptions of exclusion restriction and principal ignorability. We propose a new approach to CACE estimation, in the vein of principal stratification framework, that does not require these assumptions. We estimate the latent distribution of four relevant groups of individuals: compliers, never-takers, always-takers and defiers. We reframe the problem as a missing data problem and introduce a two-step procedure that estimates CACE via the latent distribution of the principal strata.
Céline Beji is a postdoctoral researcher at Université Paris Cité, where she collaborates with Professor Raphaël Porcher in the Personalized Medicine Team of METHODS, Inserm. Her areas of expertise include statistical machine learning and causal inference, focusing on Rubin's counterfactual framework and its applications to healthcare. Her research focuses on individual and average treatment effects, risk/benefit classification, compliance, and the use of observational data. She is also involved in deep-tech innovation and entrepreneurship.
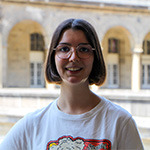
Université Paris Cité
Title: Do machine learning methods lead to similar individualized treatment rules? A comparison study on real data.
Abstract
Identifying subgroups of patients who benefit from a treatment is a key aspect of personalized medicine. Developing individualized treatment rules (ITRs), which map individual characteristics to a treatment, can be achieved by identifying these subgroups. Many machine learning algorithms have been proposed to create such rules. Yet, it is unclear to what extent those algorithms lead to the same ITRs, i.e. recommending the treatment for the same individuals. To see if methods lead to similar ITRs, we compared the most common approaches in two randomized control trials: the International Stroke Trial and the CRASH-3 trial. Two classes of methods can be distinguished to develop an ITR. The first class of methods relies on predicting individualized treatment effects from which an ITR is derived by recommending the evaluated treatment to the individuals with a predicted benefit. In the second class, methods directly estimate the ITR without estimating individualized treatment effects. The majority of the methods compared in this project fell under the first class: meta-learners (T-learner, S-learner, X-learner, DR- learner, and R-learner, both with parametric and non-parametric models), causal forests, and virtual twins, whereas A-learning, modified covariate method, outcome weighted learning and contrast weighted learning fell under the second class. When using non-parametric models, results were compared with and without cross-fitting. For each trial, the performance of ITRs was assessed in terms of value of the rule, average benefit of treatment among people with a positive score and among people with a negative score, population average prescription effect, and c-statistic for benefit. The pairwise agreement between ITRs was also calculated using Cohen’s kappa and Matthews correlation coefficients. Results showed that the ITRs obtained by the different methods generally had considerable disagreements regarding the individuals to be treated. A better concordance was found among akin methods (e.g. among all meta-learners with parametric models, or all meta-learners with non- parametric models and cross-fitting). Overall, when evaluating the performance of ITRs in a hold-out validation sample, all methods produced ITRs with limited performance, whatever the performance in the training set, which suggests a high potential for overfitting. The different methods do not lead to similar ITRs, and are, therefore, not interchangeable. The chosen method has a lot of influence on which patients end up being given a certain treatment which draws some concerns about the practical use of the methods.
Florie Bouvier is a Ph.D. candidate in Biostatistics. She has a strong focus on personalized medicine. Her research investigates the methodology for estimating individual treatment effects (ITE). It encompasses examining the performance of various statistical and machine learning techniques for the ITE estimation, utilizing data from single to multiple randomized controlled trials (RCT) through individual participant data meta-analysis.
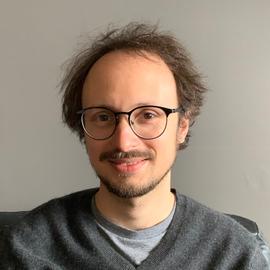
Université de Montréal, Mila
Title: Exploring Assumptions for Identifiability using Differentiable Causal Discovery Methods
Philippe Brouillard is a Ph.D. student co-supervised by Dhanya Sridhar and Alexandre Drouin at the Université de Montréal (UdeM) and Mila, the Quebec Artificial Intelligence Institute. He is currently doing an internship at ServiceNow Research. His research interests include causal discovery, causal representation learning, machine learning, and how to combine them.
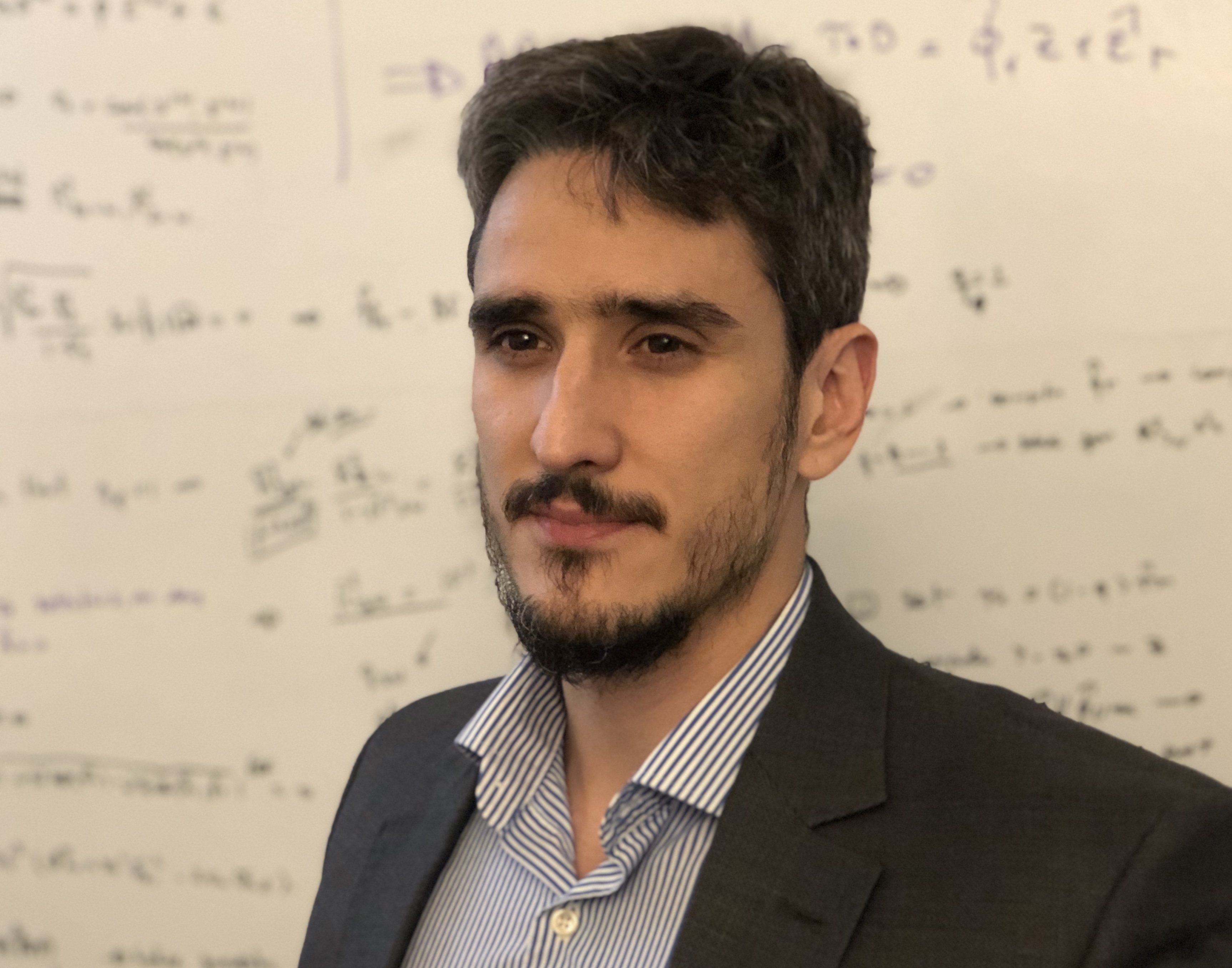
University of Washington
Title: Long Story Short: Omitted Variable Bias in Causal Machine Learning
Abstract
We derive general, yet simple, sharp bounds on the size of the omitted variable bias for a broad class of causal parameters that can be identified as linear functionals of the conditional expectation function of the outcome. Such functionals encompass many of the traditional targets of investigation in causal inference studies, such as, for example, (weighted) average of potential outcomes, average treatment effects (including subgroup effects, such as the effect on the treated), (weighted) average derivatives, and policy effects from shifts in covariate distribution -- all for general, nonparametric causal models. Our construction relies on the Riesz-Frechet representation of the target functional. Specifically, we show how the bound on the bias depends only on the additional variation that the latent variables create both in the outcome and in the Riesz representer for the parameter of interest. Moreover, in many important cases (e.g, average treatment effects and average causal derivatives) the bound is shown to depend on easily interpretable quantities that measure the explanatory power of the omitted variables. Therefore, simple plausibility judgments on the maximum explanatory power of omitted variables (in explaining treatment and outcome variation) are sufficient to place overall bounds on the size of the bias. Furthermore, we use debiased machine learning to provide flexible and efficient statistical inference on learnable components of the bounds. Finally, empirical examples demonstrate the usefulness of the approach.
Carlos Cinelli is an assistant professor at the Department of Statistics at the University of Washington. He obtained his Ph.D. in Statistics at the University of California, Los Angeles, advised by Chad Hazlett and Judea Pearl. His research focuses on developing new causal and statistical methods for transparent and robust causal claims in the empirical sciences. He is particularly interested in the inferential challenges social and health scientists face and the intersections of causality with machine learning and artificial intelligence.
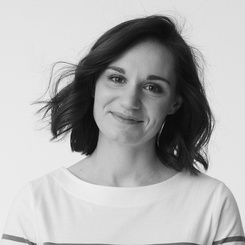
Institut Curie
Title: Analyzing the Impact of Comedications on Breast Cancer Survival: The ADRENALINE Study
Abstract
Comorbidities, existing conditions alongside cancer diagnosis, are prevalent among approximately 50% of cancer patients. These comorbidities often involve the intake of chronic medications, known as comedications. The influence of specific comedications on the long-term progression of Breast Cancer has been established by various studies. Several observational studies illustrated the influence of certain comedications on the long-term evolution of Breast Cancer. However, the analysis of the impact of comedications using observational data is a challenging causal inference task which requires extensive, high-quality datasets, and which may be prone to several types of causal biases. This presentation aims to share the findings of the ADRENALINE study, which aims to analyze the effects of comedications on breast cancer survival across the entire population of breast cancer patients in France. We will explore the causal inference framework essential for drawing meaningful conclusions, address the assumptions underlying this framework in this specific example, and discuss the advantages and limitations associated with such a comprehensive undertaking.
Elise Dumas is a Ph.D. student at RT2Lab (Residual Disease and Response to Treatment, INSERM) and CBIO (Center for Computational Biology). She is working on the effect of comedications (chronically taken medications) on survival after breast cancer using data from the SNDS (Système National des Données de Santé: French social security system).
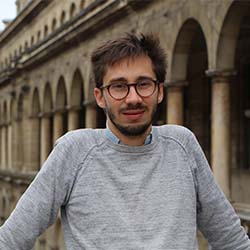
Université Paris Cité
Title: Personalizing renal replacement therapy initiation in the intensive care unit: a statistical reinforcement learning-based strategy with external validation on the AKIKI randomized controlled trials
Abstract
We used the doubly-robust estimators dWOLS on electronic health record data to learn two dynamic strategies for renal-replacement therapy (RRT) initiation in the ICU. We named these strategies “crude” and “stringent.” The major strength of our approach is its dynamic aspect: the decision rule to initiate renal replacement therapy mimics that of clinicians i.e., decisions are re-evaluated every day—for three days in a row—given patients’ evolving characteristics. We externally validated the learned strategies using the advantage double robust estimator on data from AKIKI and AKIKI 2 (two large RCTs of RRT timing). When compared to the current best practices (i.e., a standard-delayed strategy), we found that both learned strategies could improve hospital-free days at day 60. Importantly, we found that the stringent strategy could improve patients’ outcomes all the while reducing RRT prescriptions in the three days following severe AKI. This approach demonstrates how leveraging recent developments in statistics and computer science can rigorously address long outstanding clinical questions.
After training in clinical medicine, François Grolleau holds a full board certification (France) in Anesthesia and Critical Care. Then, he shifted his attention to methodological research in medicine after completing a Master of Public Health from Université Paris Descartes and a fellowship at McMaster University (Canada). He is currently an assistant professor of Biostatistics at Université Paris Cité and a researcher at CRESS — METHODS team. His scientific work focuses on developing and implementing statistical/machine learning methods for personalizing medical interventions. His applied areas of interest include Critical Care, Nephrology, and Cardiology.
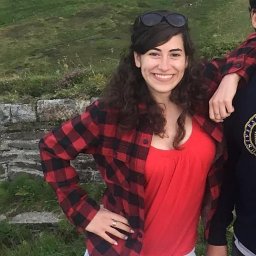
University of Oxford, The Alan Turing Institute
Title: Functional Causal Bayesian Optimization
Abstract
In this talk I will introduce the functional causal Bayesian optimization method (FCBO) for finding interventions that optimize a target variable in a known causal graph. FCBO extends CBO to perform, in addition to hard interventions, functional interventions which consist in setting a variable to be a deterministic function of a set of other variables in the graph. This is achieved by modelling the unknown objective with Gaussian processes whose inputs are defined in a reproducing kernel Hilbert space, thus allowing to compute distances among vector-valued functions. In turn, this enables to sequentially select functions to explore by maximizing an expected improvement acquisition functional while keeping the typical computational tractability of standard BO settings. We show that functional interventions can attain better target effects compared to hard interventions and ensure that the found optimal policy is also optimal for sub-groups. We demonstrate the benefits of the method in a synthetic setting and in a real-world causal graph.
Limor Gultchin is a Ph.D. student at the University of Oxford and The Alan Turing Institute. She completed her undergraduate degree in Computer Science at Harvard University and an MSc. in Social Data Science at the Oxford Internet Institute. Her research interests include causal inference and its potential impact on algorithmic transparency and accountability. Previously, she completed various projects in machine learning and natural language processing, including their use in the social sciences.
Title: Causal Inference with Information Algebras
Abstract
In a structural causal model, primitive causal relations are encoded as functional dependencies, which then map onto a graph. In this talk, we capture causality without reference to graphs or functional dependencies, but with information sigma-algebras. In the first part, we present the so-called Witsenhausen intrinsic model (WIM), originally developed for control theory. In the second part, we introduce the Information Dependency Model, as another way to handle causal relations based on the WIM. Then, we define the notion of topological separation (t-separation), which we prove to be equivalent to d-separation. We illustrate the potential of t-separation on examples.
Benjamin Heymann is a Staff Research Scientist at Criteo, interested in game theory, reinforcement learning, and causal methods. His work is motivated by applications for recommender systems and marketplace design.
Michel De Lara is a French applied mathematician trained in stochastic processes and control theory. After graduating as an engineer at Ecole Polytechnique and at Ecole Nationale des Ponts et Chaussees (ENPC), he took a research position there. Michel De Lara started his career in the environmental research center of ENPC, working part-time at the French Ministry of the Environment. He is now in a position at the mathematics research center, Cermics, where he belongs to the Optimization team. There, he addresses theoretical questions and different applications of mathematics and publishes papers in such diverse fields as biology, economics, energy, and mathematics. In his current research, Michel De Lara addresses information handling in game theory, generalized convexity, and multistage stochastic optimization. Regarding applications, aside from biodiversity management, he focuses on the management of energies in the context of fast changes in the energy system.
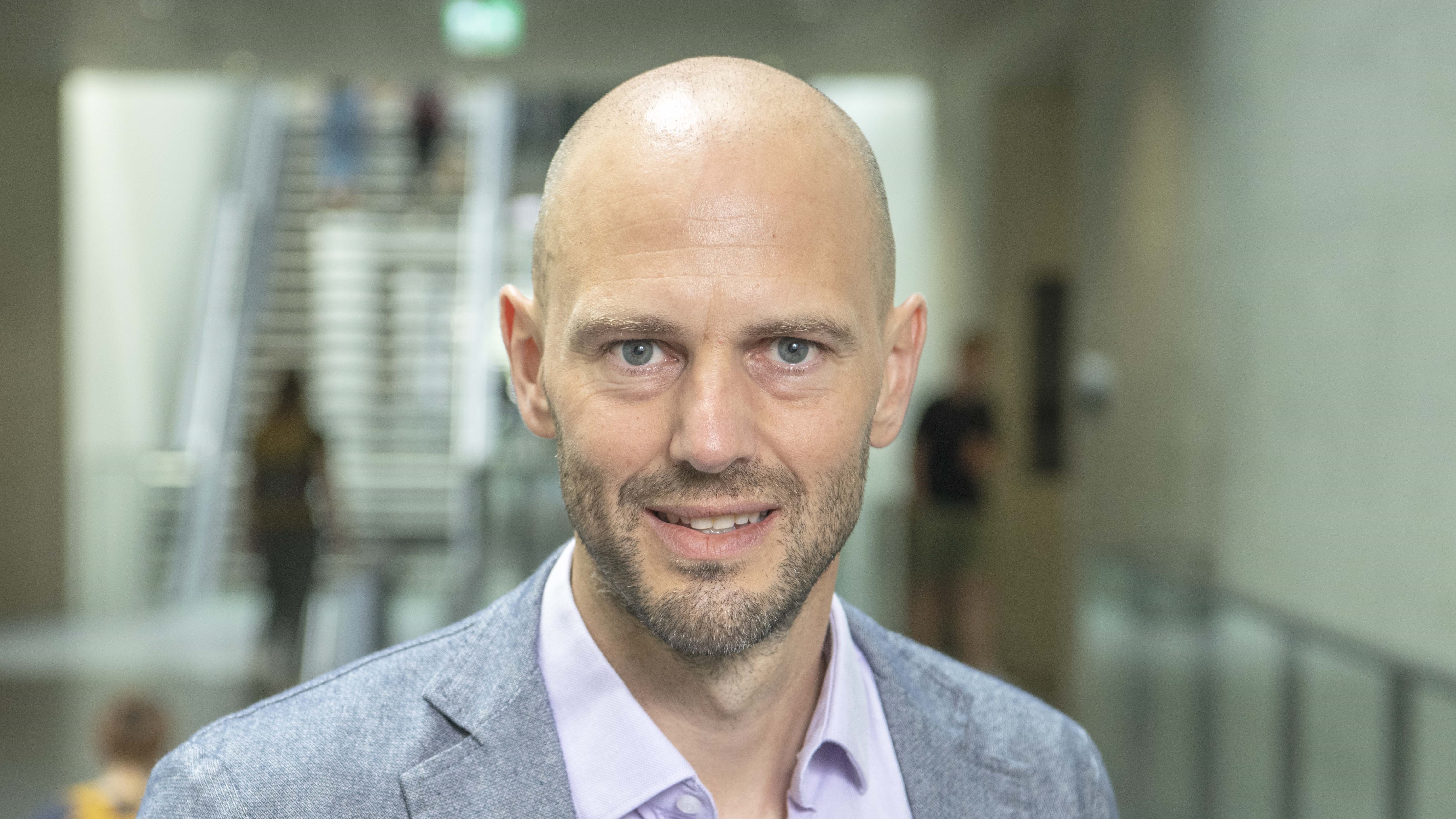
University of Fribourg
Title: Testing the identification of causal effects in observational data
Abstract
This study demonstrates the existence of a testable condition for the identification of the causal effect of a treatment on an outcome in observational data, which relies on two sets of variables: observed covariates to be controlled for and a suspected instrument. Under a causal structure commonly found in empirical applications, the testable conditional independence of the suspected instrument and the outcome given the treatment and the covariates has two implications. First, the instrument is valid, i.e. it does not directly affect the outcome (other than through the treatment) and is unconfounded conditional on the covariates. Second, the treatment is unconfounded conditional on the covariates such that the treatment effect is identified. We suggest tests of this conditional independence based on machine learning methods that account for covariates in a data-driven way and investigate their asymptotic behavior and finite sample performance in a simulation study. We also apply our testing approach to evaluating the impact of fertility on female labor supply when using the sibling sex ratio of the first two children as supposed instrument, which by and large points to a violation of our testable implication for the moderate set of socio-economic covariates considered. The paper is available here
Martin Huber is Professor of Applied Econometrics at the University of Fribourg, Switzerland, where his research comprises both methodological and applied contributions in the fields of causal analysis and policy evaluation, machine learning, statistics, econometrics, and empirical economics.
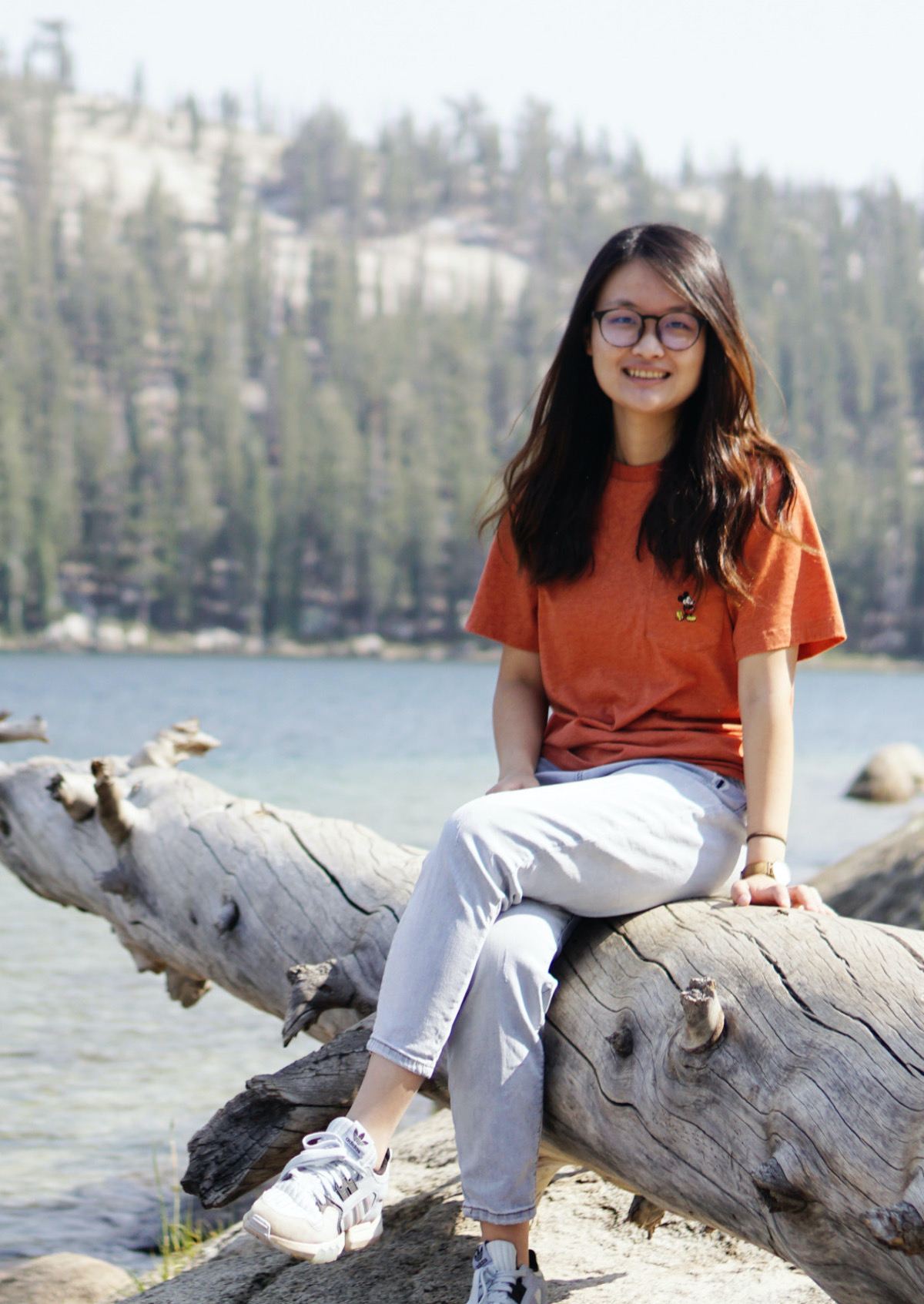
Stanford University
Title: Diagnosing the role of observed heterogeneity in replication studies
Abstract
Many researchers have identified treatment effect heterogeneity and distribution shifts as possible contributors to the reproducibility crisis in behavioral and biomedical sciences. The idea is that treatment effects that vary across individuals and contexts might be harder to detect in some populations. We propose a framework for quantifying the impact of observed heterogeneity and population discrepancy in replication studies. We decompose the difference between an original estimate and a replication estimate into "components" attributable to observed heterogeneity in baseline covariates and moderating variables, sampling variability, and residual factors. In several real-world examples from behavioral science, we find that observed heterogeneity explains little (if any) non-replicability. We discuss some implications for scientific measurement and statistical "generalizability" methods. This is joint work with Kevin Guo and Dominik Rothenhäusler.
Ying Jin is a fourth-year Ph.D. student at the Department of Statistics, Stanford University, under the supervision of Emmanuel Candès and Dominik Rothenhäusler. Her research interests include causal inference, uncertainty quantification, multiple hypothesis testing, data-driven decision-making, distributional robustness, generalizability, and replicability. Currently, she co-organizes the Online Causal Inference Seminar. She loves traveling and photography in her free time.
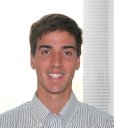
Imperial College London, Qureight
Title: Measuring axiomatic soundness of counterfactual image models
Abstract
We present a general framework for evaluating image counterfactuals. The power and flexibility of deep generative models make them valuable tools for learning mechanisms in structural causal models. However, their flexibility makes counterfactual identifiability impossible in the general case. Motivated by these issues, we revisit Pearl's axiomatic definition of counterfactuals to determine the necessary constraints of any counterfactual inference model: composition, reversibility, and effectiveness. We frame counterfactuals as functions of an input variable, its parents, and counterfactual parents and use the axiomatic constraints to restrict the set of functions that could represent the counterfactual, thus deriving distance metrics between the approximate and ideal functions. We demonstrate how these metrics can be used to compare and choose between different approximate counterfactual inference models and to provide insight into a model's shortcomings and trade-offs.
Miguel Monteiro is a machine learning engineer at Qureight. He received a Ph.D. in Computer Science from the Imperial College London in 2022 under the direction of Ben Glocker. His works mainly focus on medical imaging, uncertainty quantification, and causality.
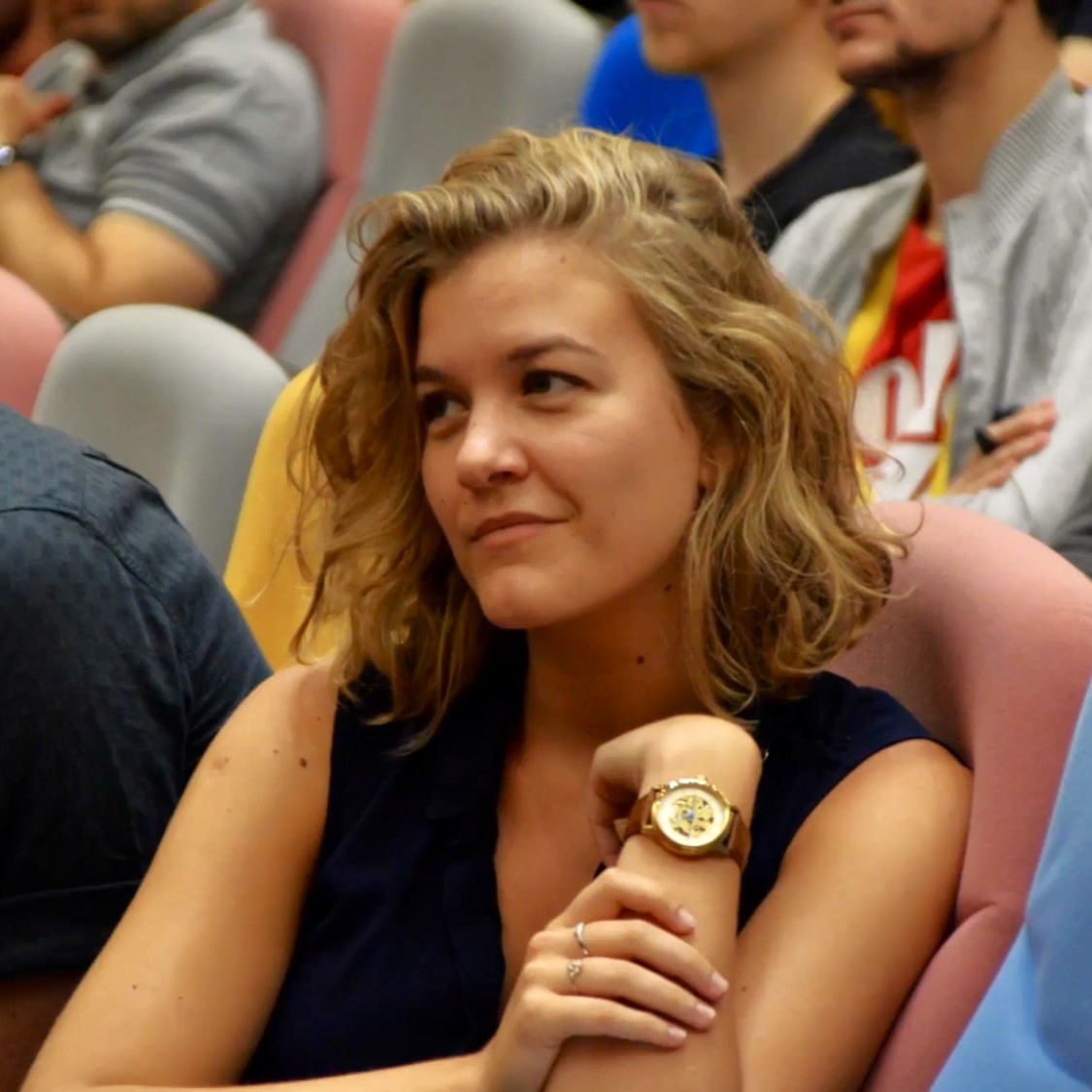
Ekimetrics, Inria, Paris-Saclay University
Title: Reconciling Mix Marketing Modelling and Causal Inference: a case study
Abstract
Marketing has embraced the causal revolution, mainly through experimentation methods such as A/B testing. However, several marketing practices based only on observational data must be combined with the causal inference approaches. It is particularly valid for Marketing Mix Modelling (MMM). Marketing Mix Modelling aims to estimate Individual Treatment Effects (ITE) of marketing activities carried out over a given period, commonly named uplift modeling. In practice, directly applying the methods developed by researchers is usually challenging because many assumptions are violated (e.g., the presence of hidden confounders and the mixture of categorical and continuous variables). Moreover, the two significant issues of MMM data are their low diversity and the high correlations of the variables (mostly spurious ones), leading marketing experts to usually make assumptions about the causal structure linking variables to interpret statistical results. This talk will discuss how causal inference can be introduced in MMM practices and improve them thanks to a Causal Data Augmentation strategy. We will discuss how modeling experts' knowledge before the estimation phase helps break down datasets' dependencies and simplifies the subsequent statistical analysis.
Audrey Poinsot is a Ph.D. student at Paris-Saclay University, the Inria TAU team, and Ekimetrics. She works on the intersection of causality and machine learning, aiming to improve decision-support tools by considering the underlying uncertainties. She has one year of experience as a consultant at Ekimetrics, where she is currently applying the results of her research on various use cases. Her research interests include causal data generation and augmentation, causal benchmarks, causal uncertainty quantification, and Trustworthy ML.
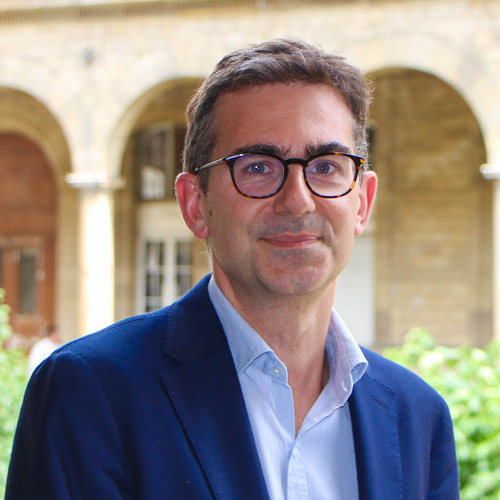
Université Paris Cité
Title: When to stop immune checkpoint inhibitor for malignant melanoma? Challenges in emulating target trials
Abstract
Observational data have become a popular source of evidence for causal effects when no randomized controlled trial exists, or to supplement information provided by those. In practice, a wide range of designs and analytical choices exist, and one recent approach relies on the target trial emulation framework. This framework is particularly well suited to mimic what could be obtained in a specific randomized controlled trial, while avoiding time-related selection biases. In this abstract, we present how this framework could be useful to emulate trials in malignant melanoma, and the challenges faced when planning such a study using longitudinal observational data from a cohort study. More specifically, two questions are envisaged: duration of immune checkpoint inhibitors, and trials comparing treatment strategies for BRAF V600-mutant patients (targeted therapy as 1st line, followed by immunotherapy as 2nd line, vs. immunotherapy as 2nd line followed by targeted therapy as 1st line). Using data from 1027 participants to the MELBASE cohort, we detail the results for the emulation of a trial where immune checkpoint inhibitor would be stopped at 6 months vs. continued, in patients in response or with stable disease.
Raphaël Porcher is a professor of Biostatistics at Université Paris Cité, and a member of the METHODS team at CRESS-UMR1153. He holds a chair at the PR[AI]RIE Artificial Intelligence Institute. He is the co-director of the Centre Virchow-Villermé. He also serves as the director of the College of Doctoral Studies at Université Paris Cité and as a member of the Comité d'Evaluation Ethique/Institutional Review Board of Inserm. With extensive expertise in biostatistics, he is actively involved in both statistical and applied clinical research, including clinical trials, observational studies, and prognostic studies. His research interests include statistical and machine learning methods for personalized medicine and methods for causal inference on treatment effects, mainly through clinical trials or observational studies. He is also involved in several international projects, including NECESSITY (NEw Clinical Endpoints in primary Sjögren's Syndrome: an Interventional Trial based on Stratifying Patients), funded by H2020-JTI-IMI2, and MORE-Europa (More Effectively Using Registries to Support Patient-centered Regulatory and HTA Decision-making), recently funded by Horizon Europe. He previously led the Work Package on Clinical Trials Designs for Personalized Medicine in the H2020-funded PERMIT (PERsonalised MedicIne Trials) project.
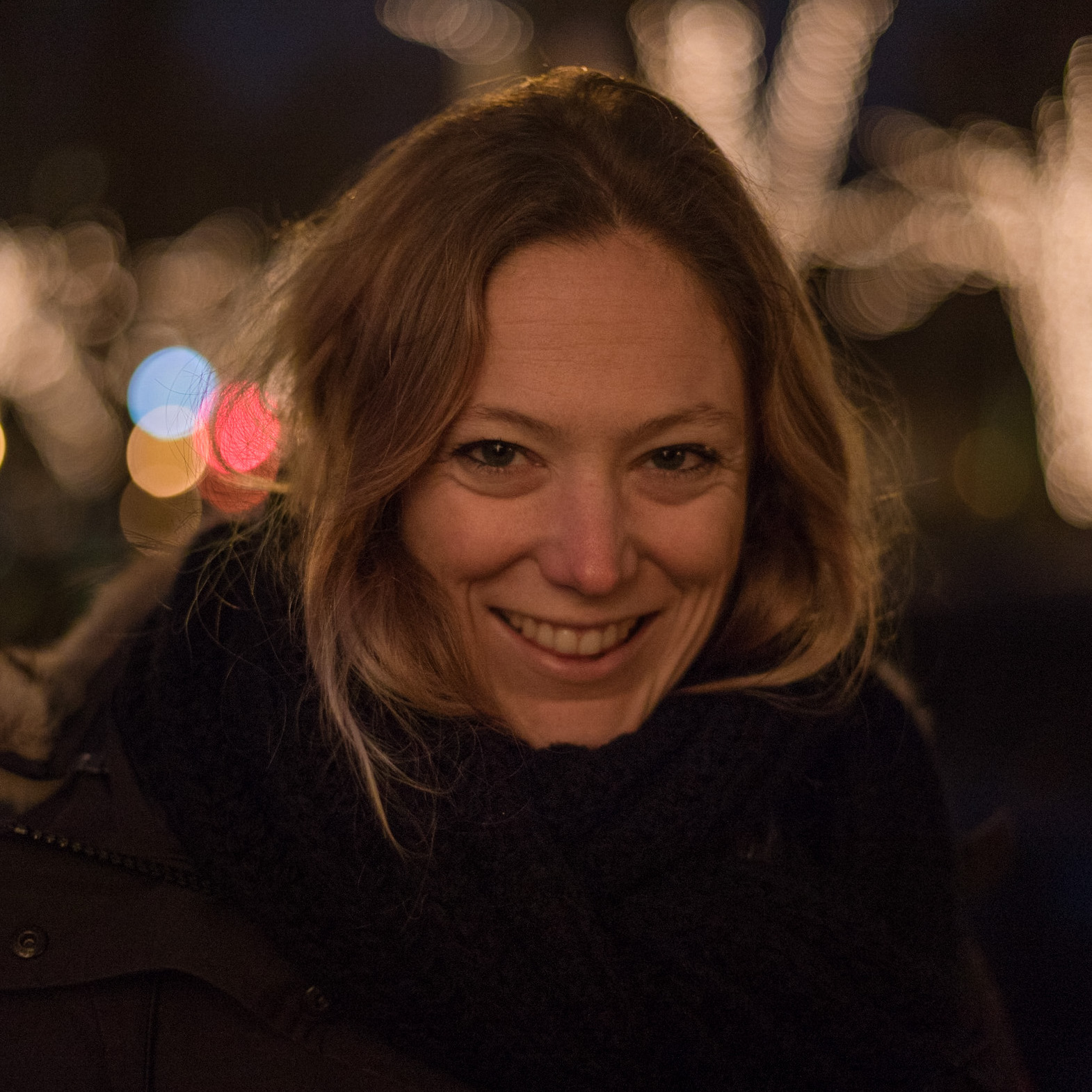
Inria
Title: New pipeline to define mechanistic correlates of protection: application to SARS-CoV-2 vaccination
Abstract
The definition of correlates of protection is critical for the development of next generation SARS-CoV-2 vaccine platforms. The complete chains of causality and interrelationships between vaccination, immune responses, protection and clinical endpoints are likely to be considerably complex. In this work, we propose a model-based approach for identifying mechanistic correlates of protection against disease acquisition based on mathematical modeling of viral dynamics and data mining of immunological markers. We apply the method to three different studies in non-human primates evaluating SARS-CoV-2 vaccines based on CD40-targeting, two-component spike nanoparticle and mRNA 1273. Inhibition of RBD binding to ACE2 appears to be a robust mechanistic correlate of protection across the three vaccine platforms although not capturing the whole biological vaccine effect.
Mélanie Prague is a permanent researcher at Inria Bordeaux Sud-Ouest Center, in the team SISTM (Statistics for Immunology and Translational Medicine, common with Inserm in Bordeaux Population Health-U1219 and Université de Bordeaux). She is responsible for the mechanistic modeling research axis of SISTM. He got a PhD in Public Health option Biostatistics at the University of Bordeaux in 2013 on the Monitoring of patients infected with HIV. Before that, She was an engineer in statistics from ENSAI and she got a master degree in mathematical statistics and econometrics. Following her thesis, she conducted a short invited researcher stay at the University of Oslo (Norway), then she was a postdoctoral fellow for 2 years and a half at Harvard School of Public Health (Boston, USA). Her work is devoted to the development and the application of statistical methods for the analysis of health data. She is particularly focused on the application of her methods to infectious diseases such as the Human Immunodeficiency Virus (HIV), Ebola, NIPAH virus and more recently SARS-CoV-2.
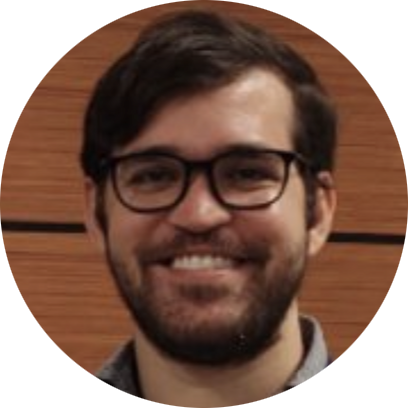
Seqera Labs
Title: Learning interpretable causal networks from observational data
Abstract
Uncovering cause-effect relationships in non-experimental settings has shown to be a very complex endeavour, given the numerous limitations and biases found in observational data. At the same time, there are many situations in which experiments cannot be performed, be it due to technical, financial or ethical reasons, and large amounts of observational data are available. Recent progress in causal discovery methodologies, and in the causal inference literature in general, has contributed to the development of techniques that learn the underlying causal structure of the events recorded through observational data, allowing us to perform causal discovery and inference in observational data. In this talk, I will go through iMIIC, a novel information-theoretic method that allows us to infer interpretable networks, and its application to a dataset of ~400,000 breast cancer patients.
Marcel Ribeiro-Dantas is a developer advocate at Seqera Labs. He holds a Ph.D. in Bioinformatics from Sorbonne Université and Institut Curie, developing causal discovery methods and investigating their application to breast cancer patients' data. He also has two graduate degrees in Big Data and Health Informatics and an MSc in Bioinformatics from the Federal University of Rio Grande do Norte (UFRN) in Brazil, where he worked on gene regulatory networks and data visualization with data from cancer patients.
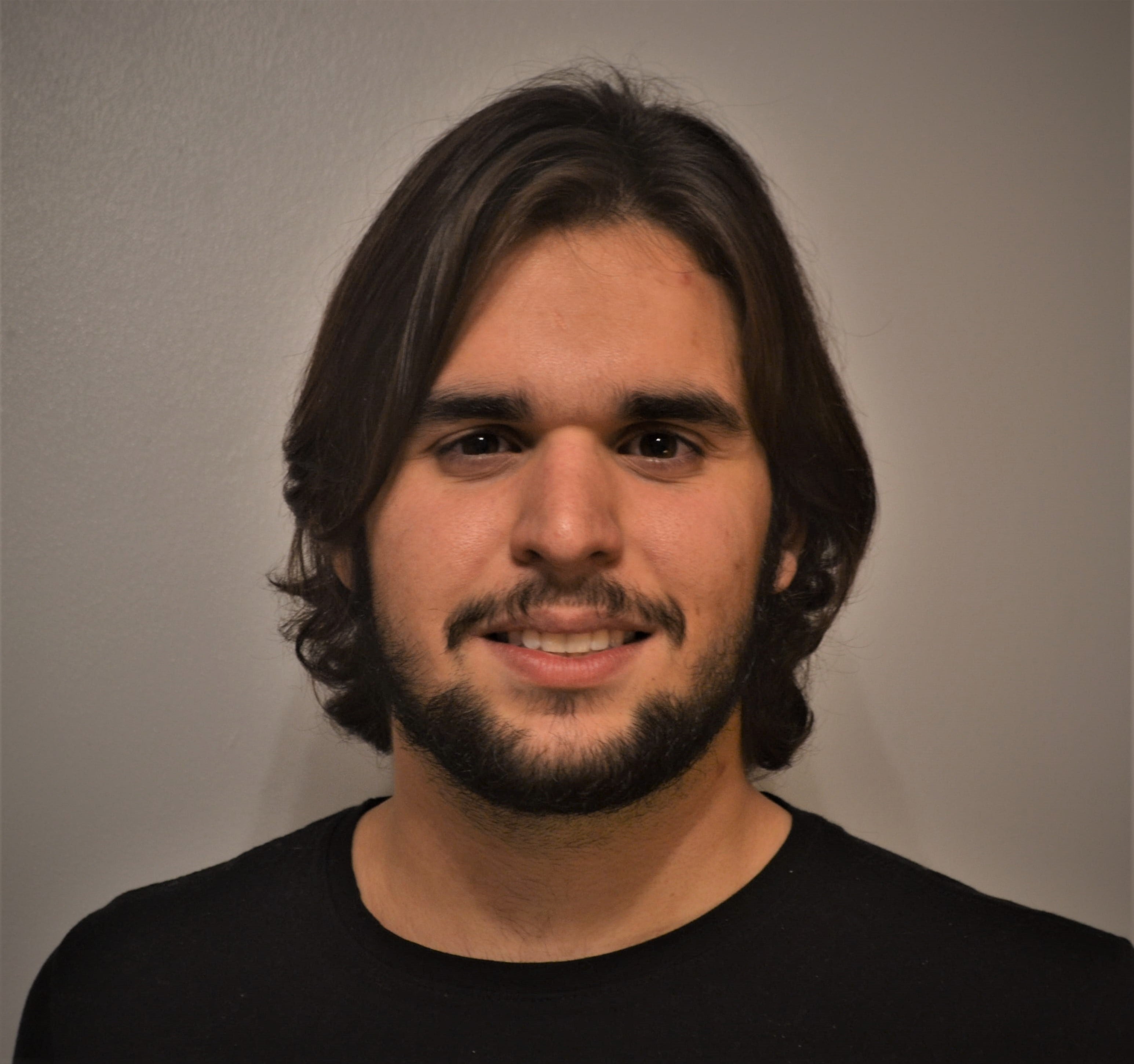
University of Edinburgh
Title: Diffusion Models for Causal Discovery via Topological Ordering
Abstract
Discovering causal relations from observational data becomes possible with additional assumptions such as considering the functional relations to be constrained as nonlinear with additive noise (ANM). Even with strong assumptions, causal discovery involves an expensive search problem over the space of directed acyclic graphs (DAGs). Topological ordering approaches reduce the optimisation space of causal discovery by searching over a permutation rather than graph space. For ANMs, the Hessian of the data log-likelihood can be used for finding leaf nodes in a causal graph, allowing its topological ordering. However, existing computational methods for obtaining the Hessian still do not scale as the number of variables and the number of samples are increased. Therefore, inspired by recent innovations in diffusion probabilistic models (DPMs), we propose DiffAN, a topological ordering algorithm that leverages DPMs for learning a Hessian function. We introduce theory for updating the learned Hessian without re-training the neural network, and we show that computing with a subset of samples gives an accurate approximation of the ordering, which allows scaling to datasets with more samples and variables. We show empirically that our method scales exceptionally well to datasets with up to nodes and up to samples while still performing on par over small datasets with state-of-the-art causal discovery methods. Implementation is available at https://github.com/vios-s/DiffAN.
Pedro Sanchez is a Ph.D. student at The University of Edinburgh, supervised by Professor Sotirios Tsaftaris and Dr. Alison O'Neil. His research focuses on the intersection between causality and machine learning applied to healthcare data. He is interested in exploring how understanding the causal structure of a problem improves generalization, merging of multi-modal information, and personalized decision-making in machine learning systems. He has more than four years of experience in (medical) image processing with deep learning during an MSc, four internships (Canon Medical, General Electric, Samsung, ICube Lab.), and working as a research engineer at Canon Medical Research Europe. He holds a Master's degree in Biomedical Engineering from the University of Strasbourg, France, and a double degree in electrical engineering and biomedical engineering from the University of Brasilia, Brazil, and the University of Strasbourg, France. Previously, He did internships at General Electric Healthcare, Samsumg Research Brazil, and ICube laboratory.
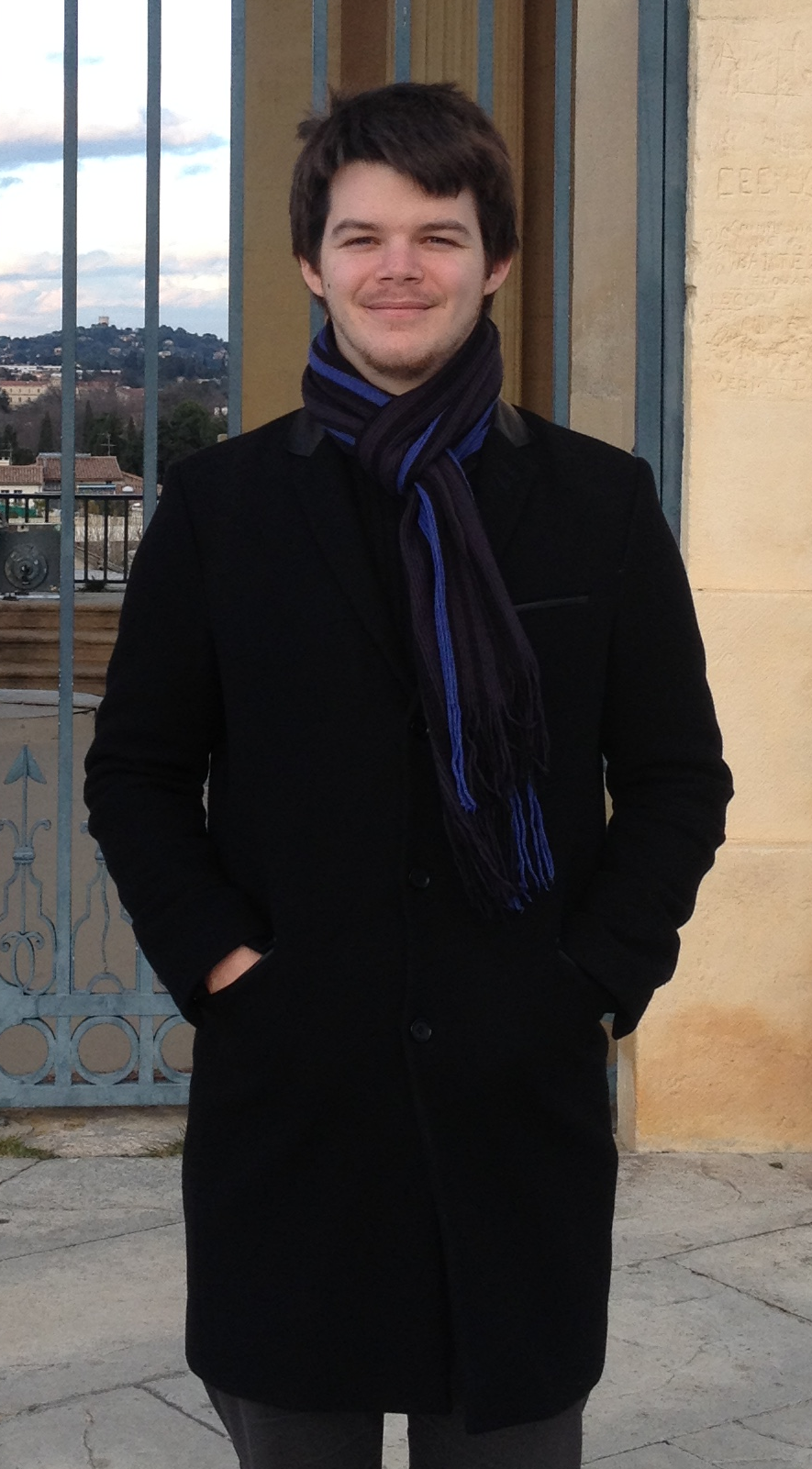
Ecole Polytechnique, CMAP
Title: From Randomized Controlled Trials to target population – a finite-sample analysis
Abstract
The limited scope of Randomized Controlled Trials (RCT) is increasingly under scrutiny, in particular when samples are unrepresentative. Indeed, some RCTs over/under-sample individuals with certain characteristics compared to the target population, for which one want to draw conclusions on treatment effectiveness. Re-weighting trial individuals to match the target population helps to improve the treatment effect estimation. Such procedures require an estimation of the ratio of the two densities (trial and target distributions). In this talk, we focus on finite-sample performances of such reweighting procedures - also called Inverse Propensity of Sampling Weighting (IPSW) - in presence of categorical covariates. We compare oracle versions of these estimates (when the trial/target distribution or the propensity score are known). Our finite-sample analysis enables us to derive precise asymptotic regimes depending on the two sample sizes (RCT and target population). In particular, we show that IPSW estimates do not benefit from using the true trial distribution if available and that IPSW performances are improved when the trial probability to be treated is estimated. In addition, we study how including covariates that are unnecessary for identifiability may impact the asymptotic variance and illustrate the results on a semi-synthetic simulation inspired from critical care medicine.
Erwan Scornet is an assistant professor at the Center for Applied Mathematics (CMAP) in Ecole Polytechnique. His research interests focus on theoretical statistics and machine learning, with a particular emphasis on nonparametric estimates. He did a Ph.D. thesis on a particular algorithm of machine learning called random forests, under the supervision of Gérard Biau (LSTA) and Jean-Philipe Vert (Institut Curie).
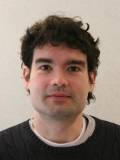
University College London
Title: Causes with many moving parts
Abstract
We can postulate a cause to be constituted of many components, some of which may not even have an well-posed way of being controlled. How will a CV land you a job? That is, to which extent does it make sense to say "my well-written CV caused me to be offered this position"? We can postulate causal meaning to the contribution of a writing clinic program for job applicants to better prepare themselves, but what is the role of the content of the document itself? Replacing words in a document may be the wrong abstraction to think about cause-effect quantification. We suggest ways by which better-posed causal models and questions can deal with such structured causes. However, even when we are lucky and each individual component of a structured cause can be individually designed, it doesn’t mean novel challenges won’t arise. How do we learn causal effects when the treatment may be something complex such as the molecular structure of a drug component? Machine learning can aid here with ideas from representation learning. Joint work with Limor Gultchin, Jean Kaddour, Matt Kusner, Qi Liu, David Watson, and Caroline Zhu.
Ricardo Silva is a Professor at the Department of Statistical Science and Adjunct Faculty of the Gatsby Computational Neuroscience Unit. Before that, Ricardo got his Ph.D. from the newly formed Machine Learning Department at Carnegie Mellon University in 2005. Ricardo also spent two years at the Gatsby Computational Neuroscience Unit as a Senior Research Fellow and one year as a postdoctoral researcher at the Statistical Laboratory in Cambridge. His research interests include machine learning, causality, graphical models, Bayesian inference, and relational inference.
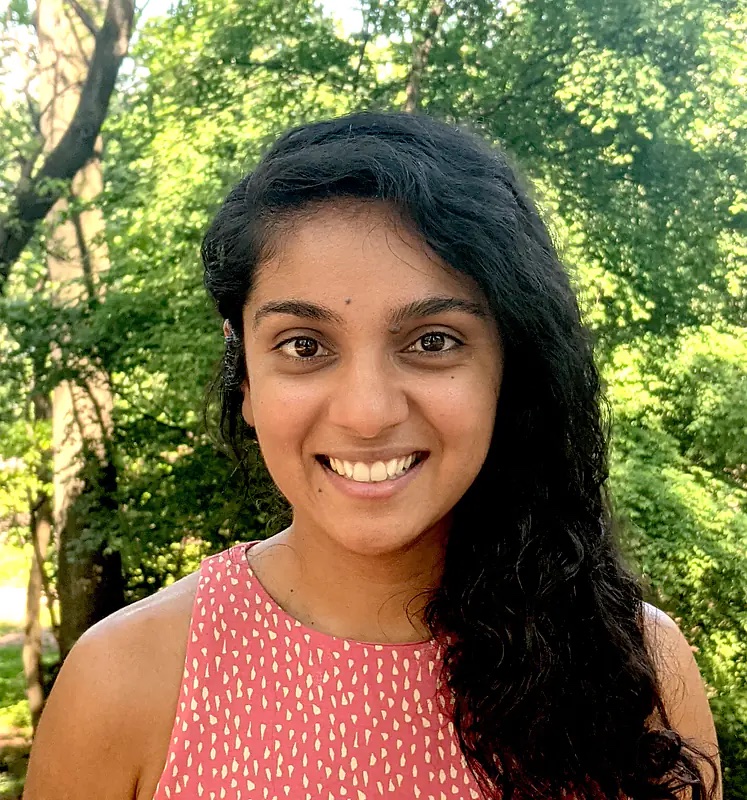
Université de Montréal and Mila
Title: Learning causal variables with machine learning
Abstract
Science and decision-making require us to infer the effects of interventions. Does knocking out a given gene suppress a function of interest? Does a proposed tax actually change some behavior of interest? Causal models provide a language to model interventions, and help us derive assumptions that yield valid causal inference. Despite the role causality plays in the sciences, the applications of causal inference have been limited, often restricted to questions where all the variables are carefully measured. In contrast, the field of machine learning (ML) has arguably succeeded at extracting task-relevant information from unstructured inputs such as text and images, inputs that implicitly capture abstract variables. Nevertheless, variables inferred using ML may not be substitutes for the underlying but unknown causal variables: ML methods may entangle the underlying causal variables, or neglect to capture them, biasing downstream causal inference. In this talk, I'll discuss two approaches to learning causally relevant variables. First, I'll introduce causally sufficient text embeddings, a general method that leverages causal model structure to learn causal variables from text data. Next, I'll discuss recent work, inspired by biological tasks, that exploits evolution in the causal mechanism mapping inputs to a target of interest to learn causal variables. Finally, I'll conclude by highlighting ongoing and open research to address the challenges of causal reasoning with ML.
Dhanya Sridhar is an assistant professor in the Department of Informatics and Operations Research (DIRO) at Université de Montréal and a core academic member of Mila. Her research focuses on combining causality and machine learning in service of AI systems that are robust to distribution shifts, adapt to new tasks efficiently, and discover new knowledge alongside us. The topics she works on span causal representation learning to robust supervised prediction. She is interested in technical results and practical algorithms that work for data such as text, images, networks, or multiple modalities.
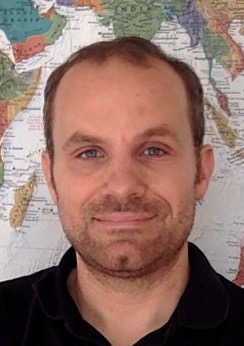
Data Science, Radboud University Nijmegen, The Netherlands
Title: Use and Testing of Causal Diagrams in Practice
Abstract
Since the publication of the landmark paper "Causal diagrams for epidemiologic research", researchers in Epidemiology and other health-related disciplines have increasingly adopted causal diagrams -- mainly to derive adjustment sets for addressing confounding bias. In the first part of this talk, I will present causal diagrams from a systematic review of DAG use in biomedical research. Unfortunately, we find the use of DAGs in practice to be rather problematic in several ways. For instance, almost no researcher appears willing to conduct any model testing to probe the consistency of their hand-drawn DAG with the dataset it is meant to represent. While there are several reasons for the lack of model testing, we hypothesize that one issue might be the difficulty of testing conditional independence (CI) statements both conceptually and in practice. Motivated by these findings, I will then discuss how the implications of causal diagrams can be tested in practice, especially in the case of mixed data. I will present existing tests for categorical data, continuous data, and ordinal data, as well as a new approach we developed that covers mixtures of all these types of data at the same time. I hope that this new testing approach will be attractive for manual testing of causal models: it is easy to implement, can be used with non-parametric or parametric statistical techniques, has an important symmetry property, and has reasonable computational cost.
Johannes Textor is an Associate Professor at Institute for Computing and Information Sciences, Radboud University. He leads the Computational Immunology group at the Data Science section of the Institute for Computing and Information Sciences at Radboud University and the Department of Tumor Immunology at the Radboud Institute for Molecular Life Sciences, Radboud University Medical Center (Radboudumc), Nijmegen, The Netherlands. He uses simulation models, machine learning, and causal inference methods to study information processing in the adaptive immune system. Likewise, his works focus on understanding how the immune system perceives and interacts with "abnormal" information from pathogens or tumors. Such knowledge helps design immunological treatments such as vaccines or tumor immunotherapies. Moreover, his works focus on developing immunologically inspired machine learning and information processing systems to understand how the immune system stores, retrieves, and modifies the information and how this differs from the second primary information-processing system in human bodies and the central nervous system.
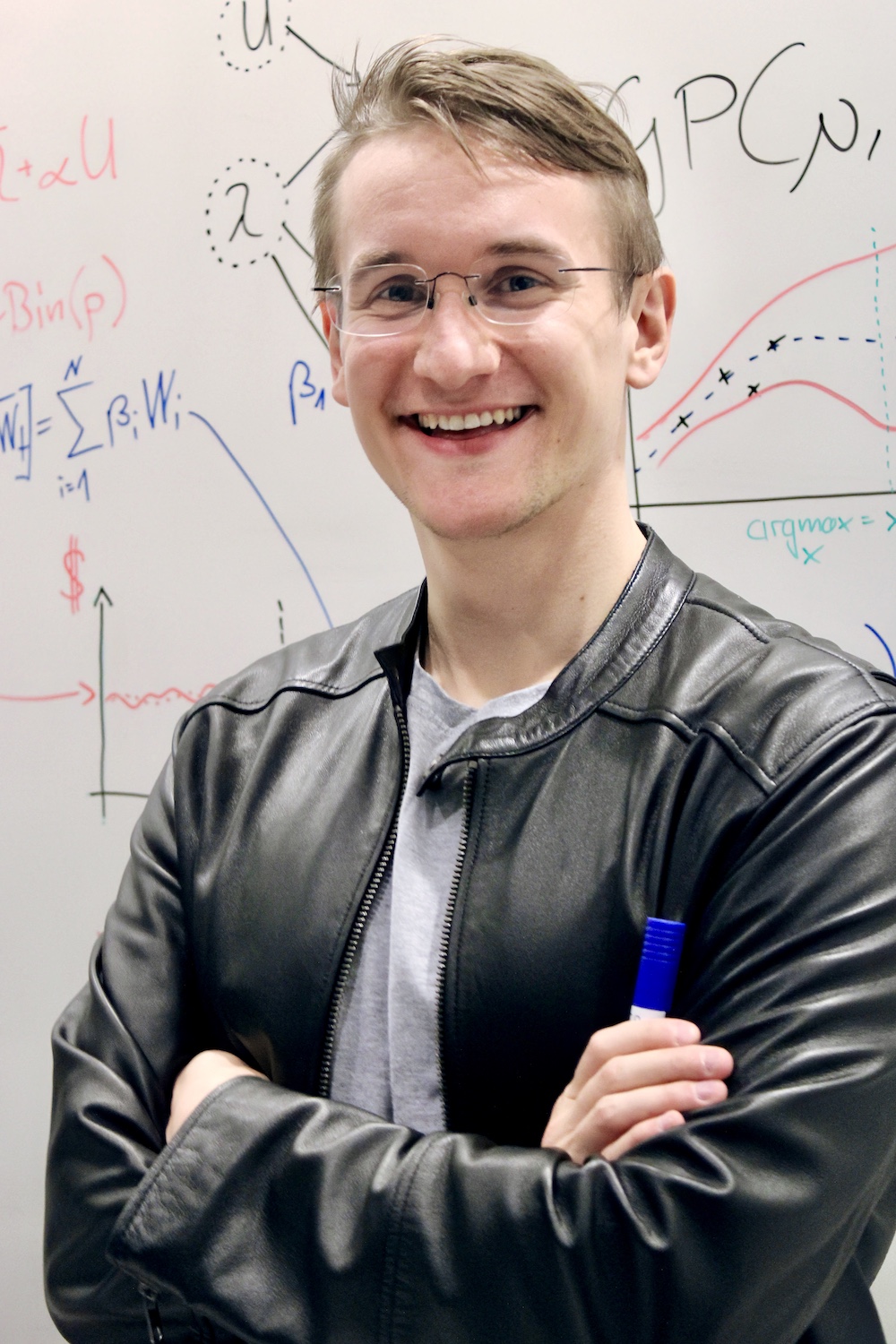
University College London
Title: Synthetic Control: Assumptions and Sensitivity Analysis for Example in Ad Campaign Evaluation
Abstract
Quantifying cause and effect relationships is an important problem in many domains. The gold standard solution is to conduct a randomised controlled trial. However, in many situations such trials cannot be performed. In the absence of such trials, many methods have been devised to quantify the causal impact of an intervention from observational data given certain assumptions. One widely used method are synthetic control models. While identifiability of the causal estimand in such models has been obtained from a range of assumptions, it is widely and implicitly assumed that the underlying assumptions are satisfied for all time periods both pre- and post-intervention. This is a strong assumption, as synthetic control models can only be learned in pre-intervention period. In this paper we address this challenge, and prove identifiability can be obtained without the need for this assumption, by showing it follows from the principle of invariant causal mechanisms. Moreover, for the first time, we formulate and study synthetic control models in Pearl's structural causal model framework. Importantly, we provide a general framework for sensitivity analysis of synthetic control causal inference to violations of the assumptions underlying non-parametric identifiability. We end by providing an empirical demonstration of our sensitivity analysis framework on simulated and real data in the widely-used linear synthetic control framework.
Jakob Zeitler is a Ph.D. student at the UCL Centre for Artificial Intelligence. His researches focus on methods and limitations of causal inference and their intersection with machine learning. He believes causal inference can only work in the real world if we are honest about its assumptions. He is looking at these problem settings with trustworthy properties, including partial identification, causal Bayesian optimization, and topological perspectives of causal inference.
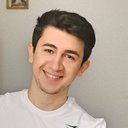
TU Darmstadt
Title: Large Language Models and Causality: Like Parrots Mimicking the Words of Humans
Abstract
In this session we will try to explore together both the capabilities and ultimate limitations of large language models (LLMs) when it comes to causal inference. While some researchers argue that scale is all what is needed to achieve AI, covering even causal models, throughout our session it will become clear that the all-scaled-up LLMs cannot be causal and we will give reason onto why sometimes we might feel otherwise when interacting with them. We conjecture that in the cases were LLM indeed succeed in doing causal inference, underlying was a respective meta SCM that exposed correlations between causal facts in natural language on whose data the LLM was ultimately trained. If our hypothesis holds true, then this would imply that LLMs are like parrots in that they simply recite the causal knowledge embedded in the data. Put differently: just knowing, not understanding.
Matej Zečević is a Ph.D. Candidate in Computer Science at the Artificial Intelligence & Machine Learning Lab (TU Darmstadt) under Prof. Kristian Kersting. He contributes to System 2 AI by unifying Causality with AI/ML, "as graphs are for causation, causal nets are for AI."
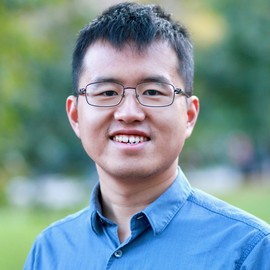
University of Cambridge
Title: Almost exact Mendelian randomization
Abstract
Mendelian randomization (MR) is a natural experimental design based on the random transmission of genes from parents to offspring. However, this inferential basis is typically only implicit or used as an informal justification. As parent-offspring data becomes more widely available, we advocate a different approach to MR that is exactly based on this natural randomization, thereby formalizing the analogy between MR and randomized controlled trials. We begin by developing a causal graphical model for MR which represents several biological processes and phenomena, including population structure, gamete formation, fertilization, genetic linkage, and pleiotropy. This causal graph is then used to detect biases in population-based MR studies and identify sufficient confounder adjustment sets to correct these biases. We then propose a randomization test in the within-family MR design using the exogenous randomness in meiosis and fertilization, which is extensively studied in genetics. Besides its transparency and conceptual appeals, our approach also offers some practical advantages, including robustness to misspecified phenotype models, robustness to weak instruments, and elimination of bias arising from population structure, assortative mating, dynastic effects, and horizontal pleiotropy. We conclude with an analysis of a pair of negative and positive controls in the Avon Longitudinal Study of Parents and Children.
Qingyuan Zhao is an Assistant Professor in the Statistical Laboratory, Department of Pure Mathematics and Mathematical Statistics (DPMMS) at University of Cambridge. He is a Fellow of the Corpus Christi College, and of the Alan Turing Institute. He is interested in improving the general quality and appraisal of statistical research, including new methodology and a better understanding of causal inference, novel study designs, sensitivity analysis, multiple testing, and selective inference.
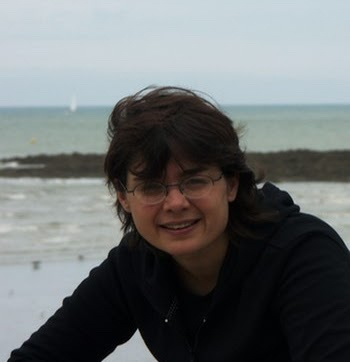
University of Lorraine
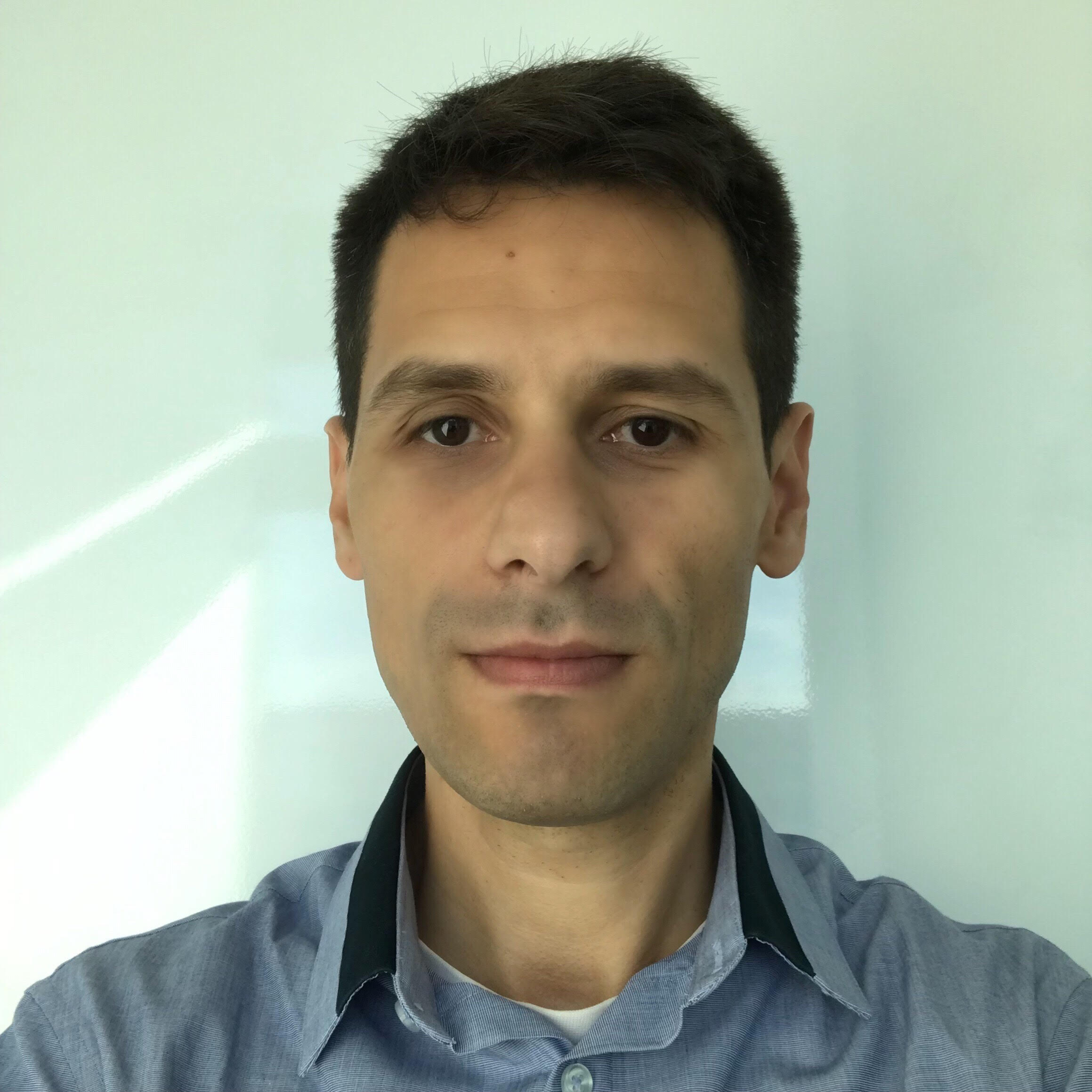
TAU, INRIA, Paris-Saclay University
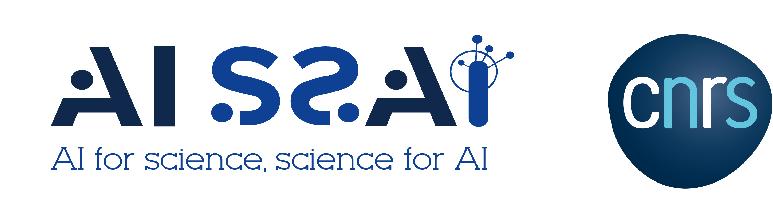
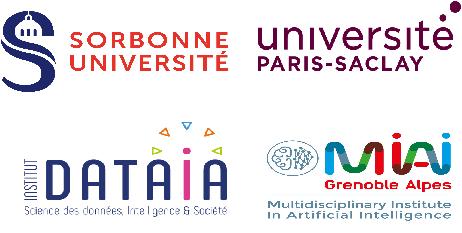
Code of Conduct
Our Causality in Practice is dedicated to providing a harassment-free experience for everyone, regardless of gender, gender identity and expression, age, sexual orientation, disability, physical appearance, body size, race, ethnicity, religion (or lack thereof), or technology choices. We do not tolerate harassment of participants in any form. Sexual language and imagery is not appropriate for any venue, including talks, workshops, parties, Twitter and other online media. Participants violating these rules may be sanctioned or expelled from the event at the discretion of the conference organizers. If you have any concerns about possible violation of the policies, please contact the organizers (organizers.quarter.causality@gmail.com) as soon as possible.