Thematic Quarter on Causality - Opening Session
Institute Henri Poincaré, Paris, France
April 11th, 2023
Location: 11 Rue Pierre et Marie Curie, 75005 Paris, France
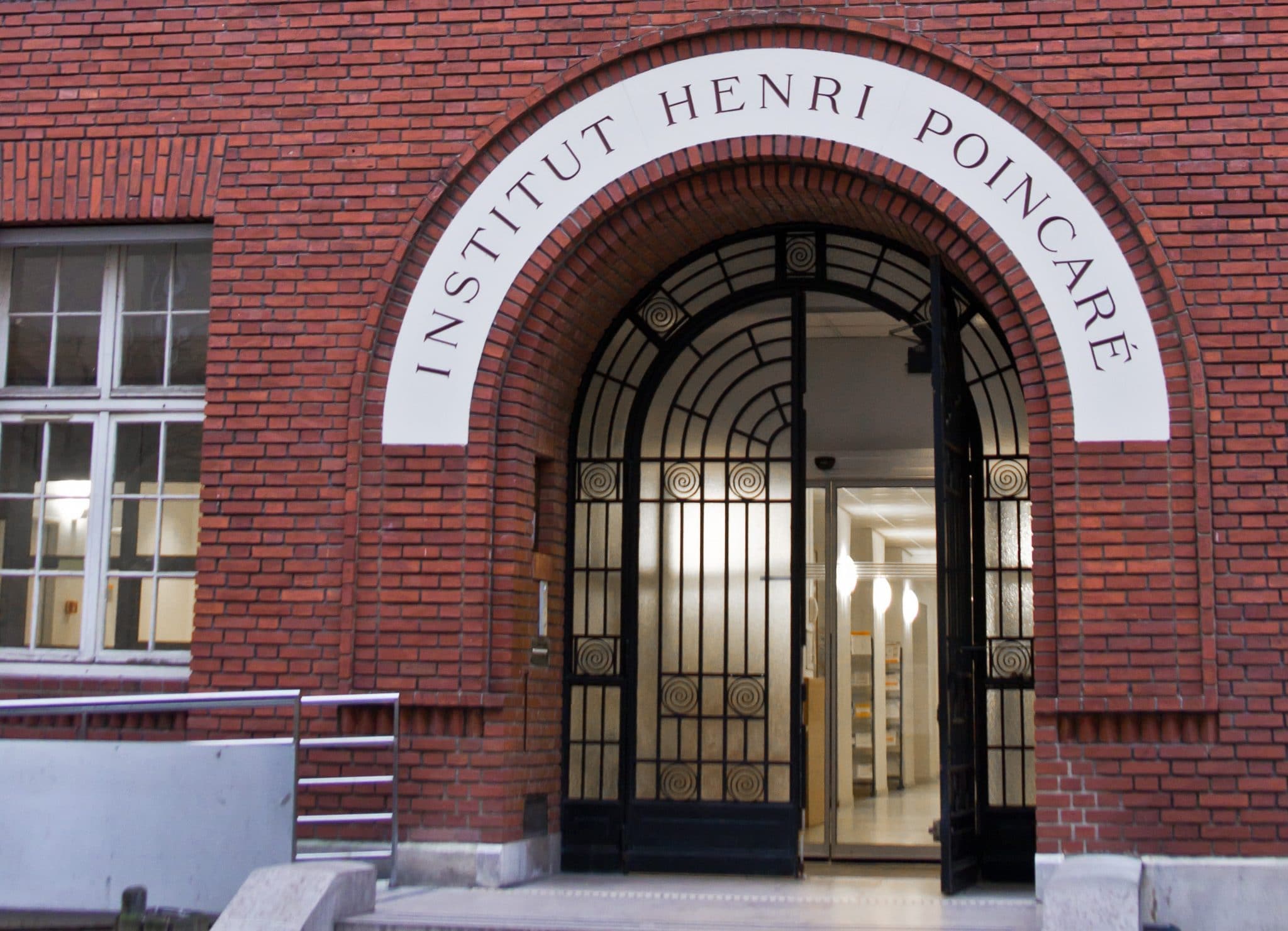
Registration
Participation is free of charge, but registration is mandatory.
Please register here before April 7th AoE.
All the talks will be at the Auditorium Hermite.
The conference will be broadcast here
Join the Webex meeting here to ask questions online.
- Meeting number (access code): 2740 402 6513
- Meeting password: RKj6RBUav22
Schedule
Tuesday, April 11th | |
---|---|
9:00 AM - 9:30 AM | Registration & welcome coffee |
9:30 AM - 9:45 AM | Welcome |
10:00 AM - 11:00 AM | Keynote by David Blei |
11:00 AM - 11:30 PM | Coffee break |
11:30 AM - 12:30 PM | Keynote by Jonas Peters |
12:30 PM - 1:45 PM | Lunch & Poster Session (buffet served on the conference premises) |
1:45 PM - 2:45 PM | Keynote by Nataliya Sokolovska |
2:45 PM - 3:45 PM | Keynote by Caleb H. Miles |
3:45 PM - 4:15 PM | Keynote by Antoine Bertoncello |
4:15 PM - 4:30 PM | Coffee break |
4:30 PM - 5:15 PM | Keynote by Thomas Richardson |
5:15 PM - 6:00 PM | Round-table discussion |
Keynote Speakers
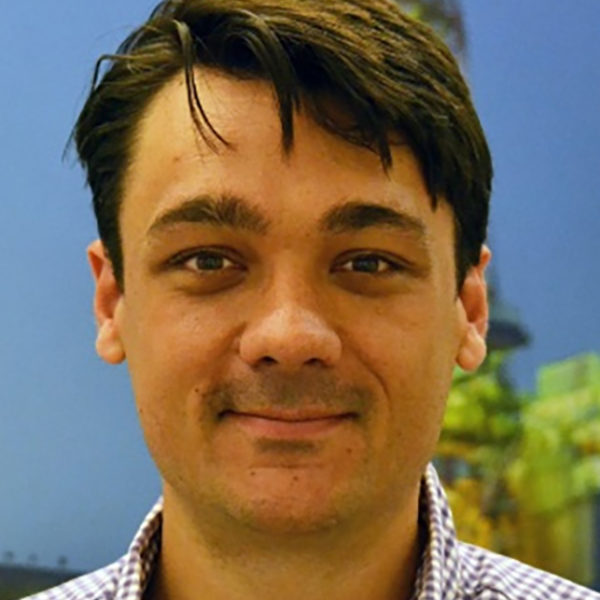
TotalEnergies
Title: Beyond Correlation: Causal Inference at TotalEnergies
Abstract
Causal inference is crucial for answering complex questions across various fields but has seen limited adoption in industrial settings. This talk explores TotalEnergies' efforts in applying causal inference to their operations, discussing the potential application in TotalEnergies and the technical challenges we encountered.
Antoine Bertoncello is the head of Next Generation AI at TotalEnergies. He holds a doctoral degree from Stanford University and Engineering degree from ENSG-Nancy. At TotalEnergies, his team aims to develop causal discovery and inference methods for renewable energy applications.
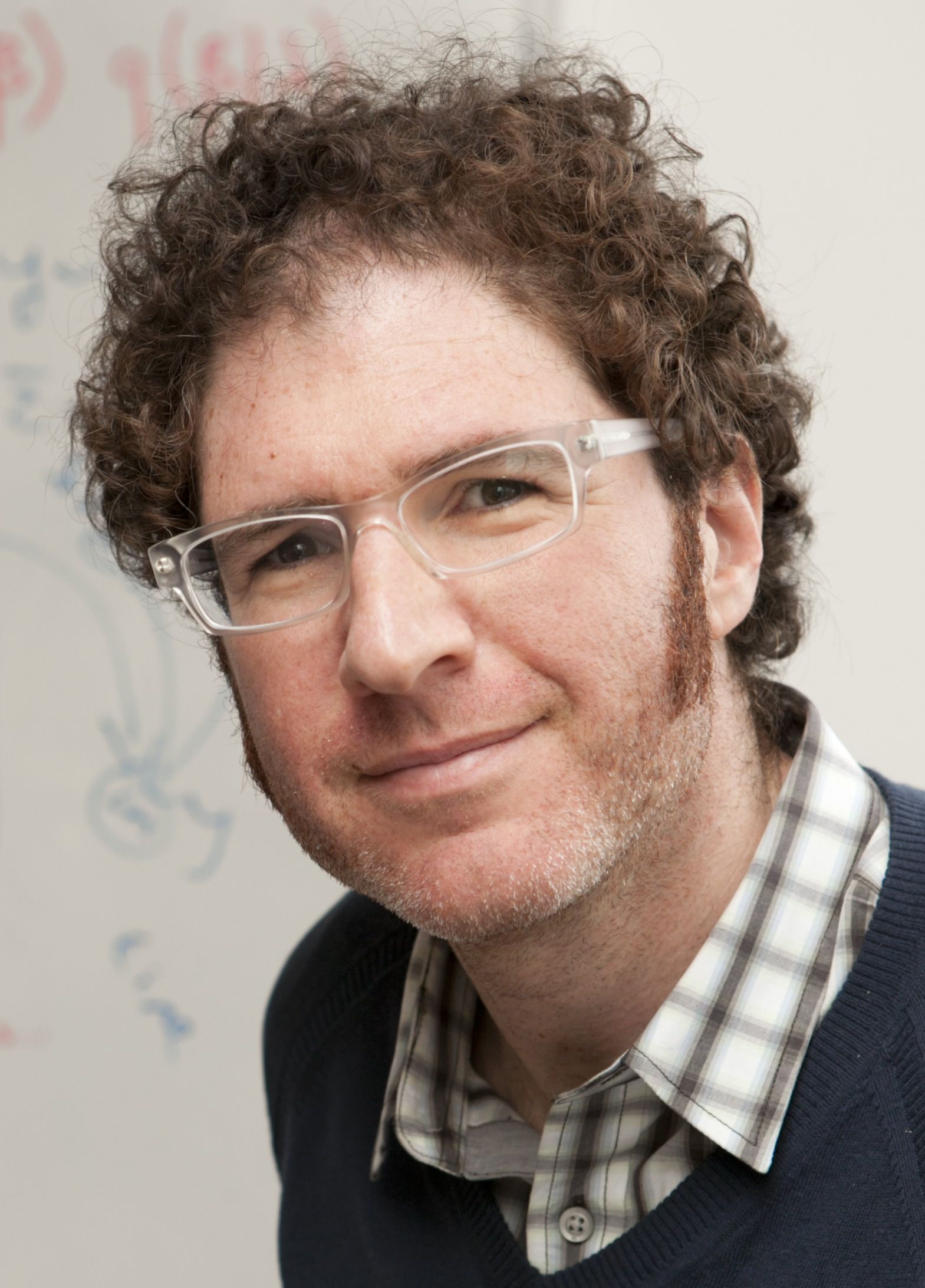
Columbia University
Title: Hierarchical Causal Models
Abstract
Analyzing nested data with hierarchical models is a staple of Bayesian statistics, but causal modeling remains largely focused on "flat" models. In this talk, we will explore how to think about nested data in causal models, and consider the advantages of nested data over aggregate data (such as data means) for causal inference. We show that disaggregating your data---replacing a flat causal model with a hierarchical causal model---can provide new opportunities for identification and estimation. As examples, we will study how to identify and estimate causal effects under unmeasured confounders, interference, and instruments. (This is a joint work with Eli Weinstein.)
David Blei is a professor of Statistics and Computer Science at Columbia University. He is also a member of the Columbia Data Science Institute. He works primarily in the fields of machine learning and Bayesian statistics.
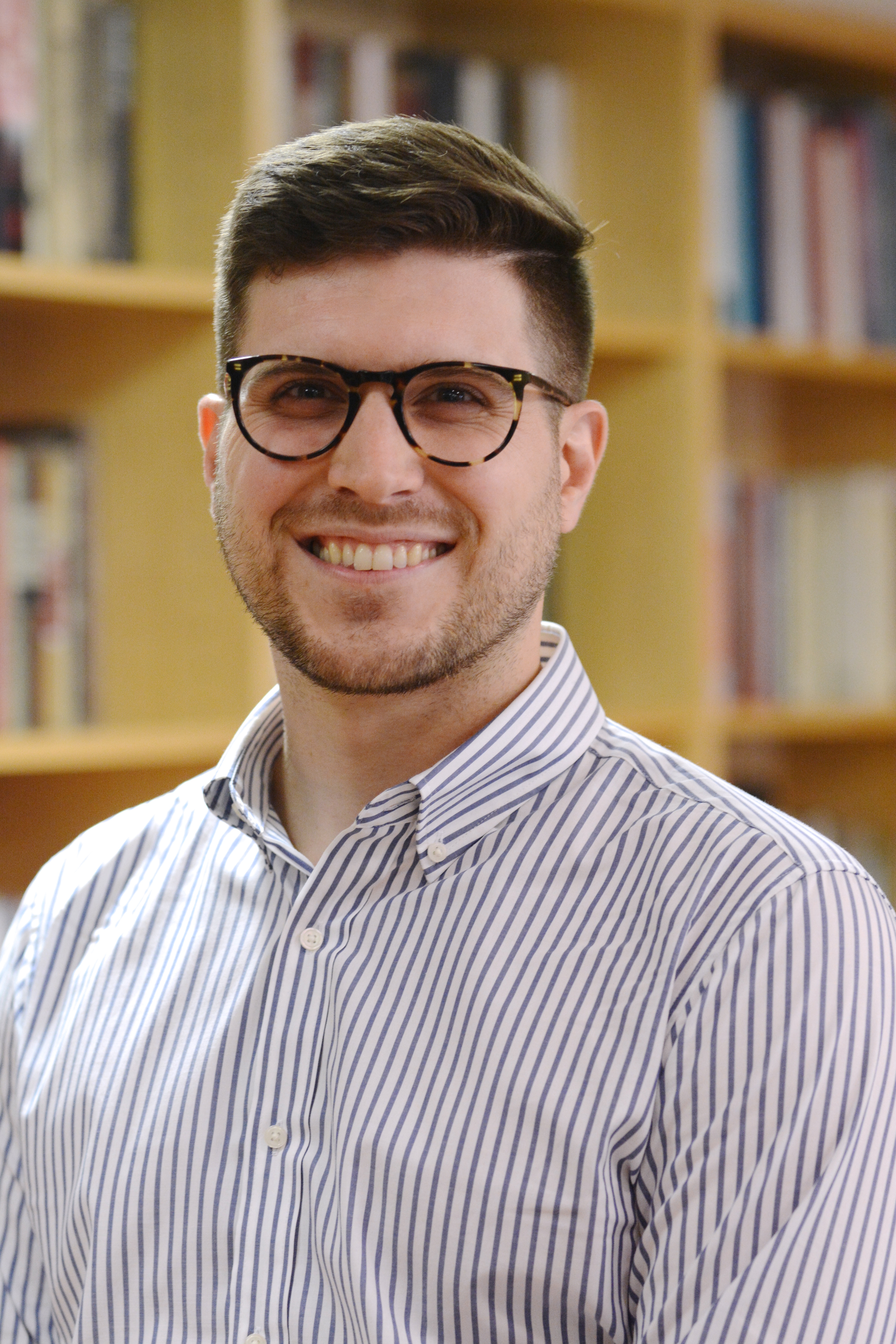
Columbia University Mailman School of Public Health
Title: Some fundamental problems in causal mediation analysis
Abstract
Scientists are often interested in understanding mediating mechanisms that can help explain causal effects. A vast body of literature on mediation analysis has accumulated since two foundational articles (Robins and Greenland, 1992; Pearl, 2001) formalized mediation in the language of causality. However, causal mediation analysis poses many fundamental and interesting difficulties in its causal interpretation, nonparametric identification assumptions (which are much stronger than most typical causal assumptions), and statistical inference. I will discuss some of my work in each of these categories: (i) the interpretation of alternative estimands that have been proposed to circumvent the usual identification assumptions, (ii) the implicit assumptions embedded in the standard mediation identification assumptions and their shortcomings in handling the inherent time-varying nature of mediators, and (iii) the well-known issue of the conservative and underpowered nature of hypothesis testing for mediated effects.
Caleb H. Miles is an assistant professor in the Department of Biostatistics at the Columbia University Mailman School of Public Health. He works on developing semi-parametric methods for causal inference and applying them to problems in public health. His applied work has largely been in HIV/AIDS, and he has more recently begun to work on psychiatric applications. His current methodological research interests include causal inference, its intersection with machine learning, mediation analysis, interference, and measurement error.
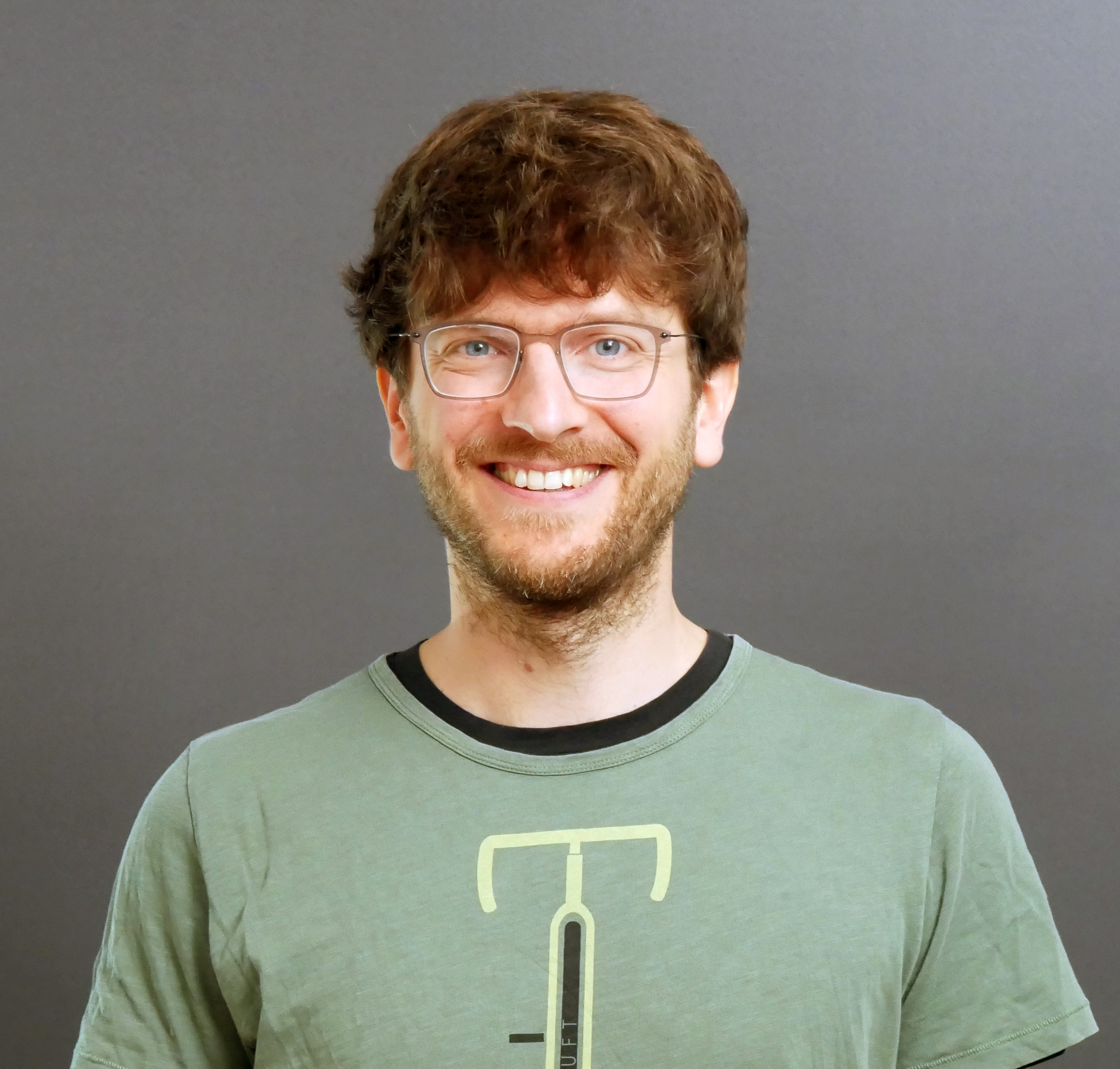
ETH Zurich
Title: More covariates than instruments -- what can we do?
Abstract
Exogenous heterogeneity, for example in the form of instrumental variables, can help us learn a system's underlying causal structure and predict the outcome of unseen intervention experiments. In this talk, we discuss this idea in a setting in which the causal effect from covariates on the response is generally not identifiable as there may be more covariates than instruments, for example.
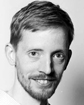
University of Washington
Title: Single World Intervention Graphs
Abstract
A simple framework for unifying causal graphs and potential outcomes with applications to mediation analysis Joint work with J.M. Robins (Harvard) and I. Shpitser (Johns Hopkins)
Thomas Richardson is a professor in the Department of Statistics. He is also an adjunct professor in the Departments of Economics and Electrical Engineering and a member of the eScience Steering Committee. He received his BA from the University of Oxford and his MS and PhD from Carnegie Mellon University. He is a Fellow of the Center for Advanced Studies in the Behavioral Sciences at Stanford University. His research interests include Graphical Models and Causality.
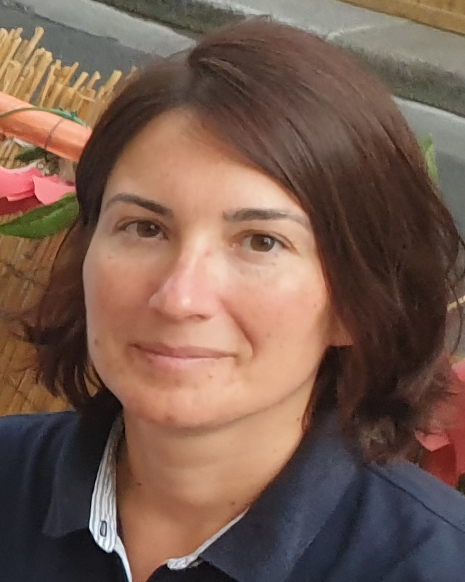
Sorbonne University
Title: The role of instrumental variables in causal inference
Abstract
Causal inference methods based on conditional independence construct Markov equivalent graphs, and cannot be applied to bivariate cases. The approaches based on independence of cause and mechanism state, on the contrary, that causal discovery can be inferred for two observations. We challenge to reconcile these two research directions. We study the role of latent variables such as latent instrumental variables and hidden common causes in the causal graphical structures. We show that the methods based on the independence of cause and mechanism indirectly contain traces of the existence of the hidden instrumental variables. I will present our novel algorithm to infer causal relationships between two variables, which was validated on simulated data and on a benchmark of cause-effect pairs.
Nataliya Sokolovska is a full professor at Sorbonne University. She holds a doctoral degree from Telecom ParisTech. Her research interests include graphical models, probabilistic inference, causal inference, interpretable models, semi-supervised learning, reinforcement learning, and game theory.
Posters
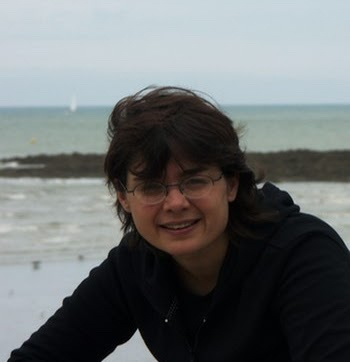
University of Lorraine
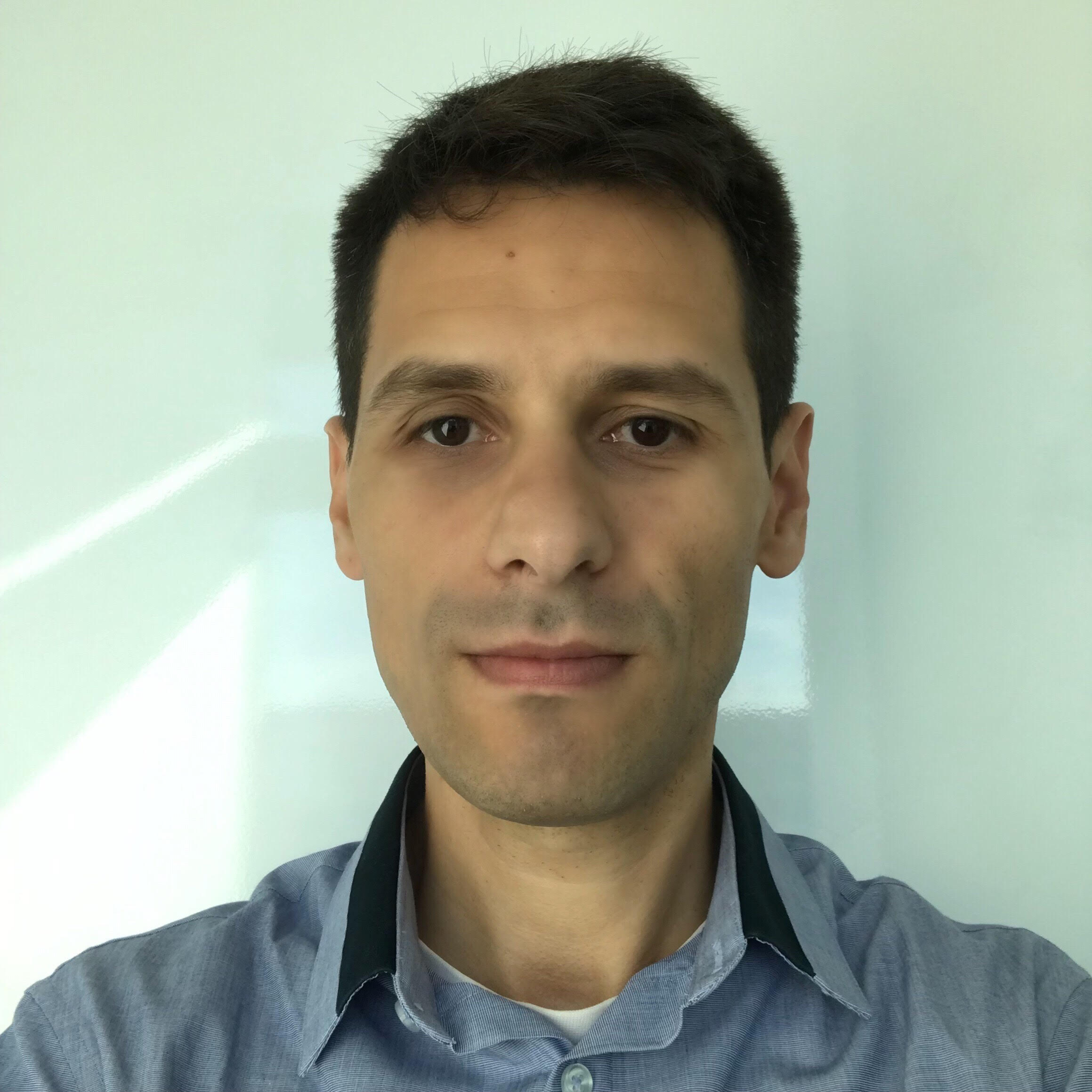
TAU, INRIA, Paris-Saclay University
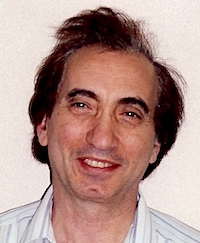
Paris-Saclay University
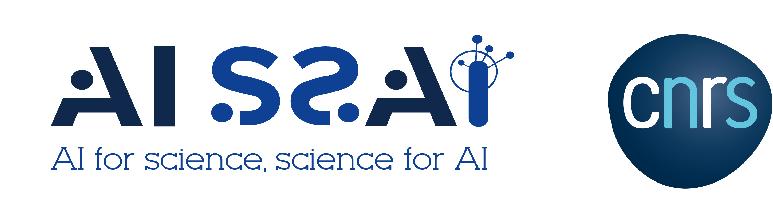
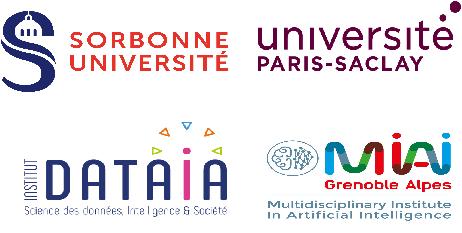
Code of Conduct
Our When Causal Inference meets Statistical Analysis is dedicated to providing a harassment-free experience for everyone, regardless of gender, gender identity and expression, age, sexual orientation, disability, physical appearance, body size, race, ethnicity, religion (or lack thereof), or technology choices. We do not tolerate harassment of participants in any form. Sexual language and imagery is not appropriate for any venue, including talks, workshops, parties, Twitter and other online media. Participants violating these rules may be sanctioned or expelled from the event at the discretion of the conference organizers. If you have any concerns about possible violation of the policies, please contact the organizers (organizers.quarter.causality@gmail.com) as soon as possible.