Fundamental Challenges in Causality
Grenoble Alpes University, Grenoble, France
From May 9th to May 12th

The colloquium on Fundamental Challenges on Causality (FunCausal) aims to bring together researchers interested in causality and willing to discuss novel approaches to causal discovery and causal inference. FunCausal will explore topics related to, but not limited to:
- Causal discovery
- Causal learning and control problems
- Theoretical foundation of causal inference
- Causal inference and active learning
- Causal learning in low data regime
- Reinforcement learning
- Causal machine learning
- Causal generative models
- Benchmark for causal discovery and causal reasoning
Venue
The colloquium will take in the auditorium IMAG building of the University located in Saint-Martin-d'Hères (next to Grenoble). Detailed information on how to reach this building from various places can be found here. In case of problem, please contact E. Gaussier (+33 682 197 988).Attending remotely
You can follow the presentations online via: https://univ-grenoble-alpes-fr.zoom.us/j/94684362046?pwd=dWl1bDdvZXZjcnVGaXlJSU9SdmdXdz09 (id: 946 8436 2046; code: 938735).Program
Tuesday 9 May | |
---|---|
2:00pm - 2:15pm | Opening remarks |
2:15pm - 3:45pm | Keynote by R. Evans (with Xi Lin) - Combining Randomized and Observational Studies (Combine Randomized and Observational Data through a Power Likelihood) |
3:45pm - 4:15pm | Coffee break |
4:15pm - 5:30pm | Round table - Causality: What does it mean? In theory? In practice? |
5:30pm - 6:30pm | Cocktail & Posters |
Wednesday 10 May | |
9:00am - 10:30am | Keynote by M. Sébag - Causal Modeling through (i) Adversarial Learning and (ii) Inverse Covariance Matrix Decomposition |
10:30am - 11:00am | Coffee break & posters |
11:00am - 12:30pm | Oral presentations
|
12:30pm - 2:00pm | Lunch (buffet served on the conference premises) |
2:00pm - 3:30pm | Keynote by J. Runge (with Urmi Ninad) - Causal Inference for Complex Spatio-Temporal Systems |
3:30pm - 4:00pm | Coffee break & posters |
4:00pm - 5:30pm | Keynote by I. Shpitser (with Amiremad Ghassami) - The Proximal ID Algorithm |
Thursday 11 May | |
9:00am - 10:30am | Keynote by H. Isambert (with Louise Dupuis) - Reliable Causal Discovery from Information Theoretic Principles |
10:30am - 11:00am | Coffee break & posters |
11:00am - 12:30pm | Round table: What's the place of causality in ML? |
12:30pm - 2:00pm | Lunch (buffet served on the conference premises) |
2:00pm - 3:30pm | Keynote by M. van der Schaar (with Jeroen Berrevoets) - Causal Deep Learning |
3:30pm - 4:00pm | Coffee break & posters |
4:00pm - 5:30pm | Oral presentations
|
7:30pm - 10:30pm | Dinner |
Friday 12 May | |
9:00am - 10:30am | Keynote by K. Zhang - Causal Representation Learning: Successes and Challenges |
10:30am - 10:45am | Coffee break & Posters |
10:45am - 11:30am | Oral presentations
|
11:30am - 12:00pm | Discussion & conclusion |
12:30pm - 2:00pm | Lunch (buffet served on the conference premises) |
Invited Keynote Speakers
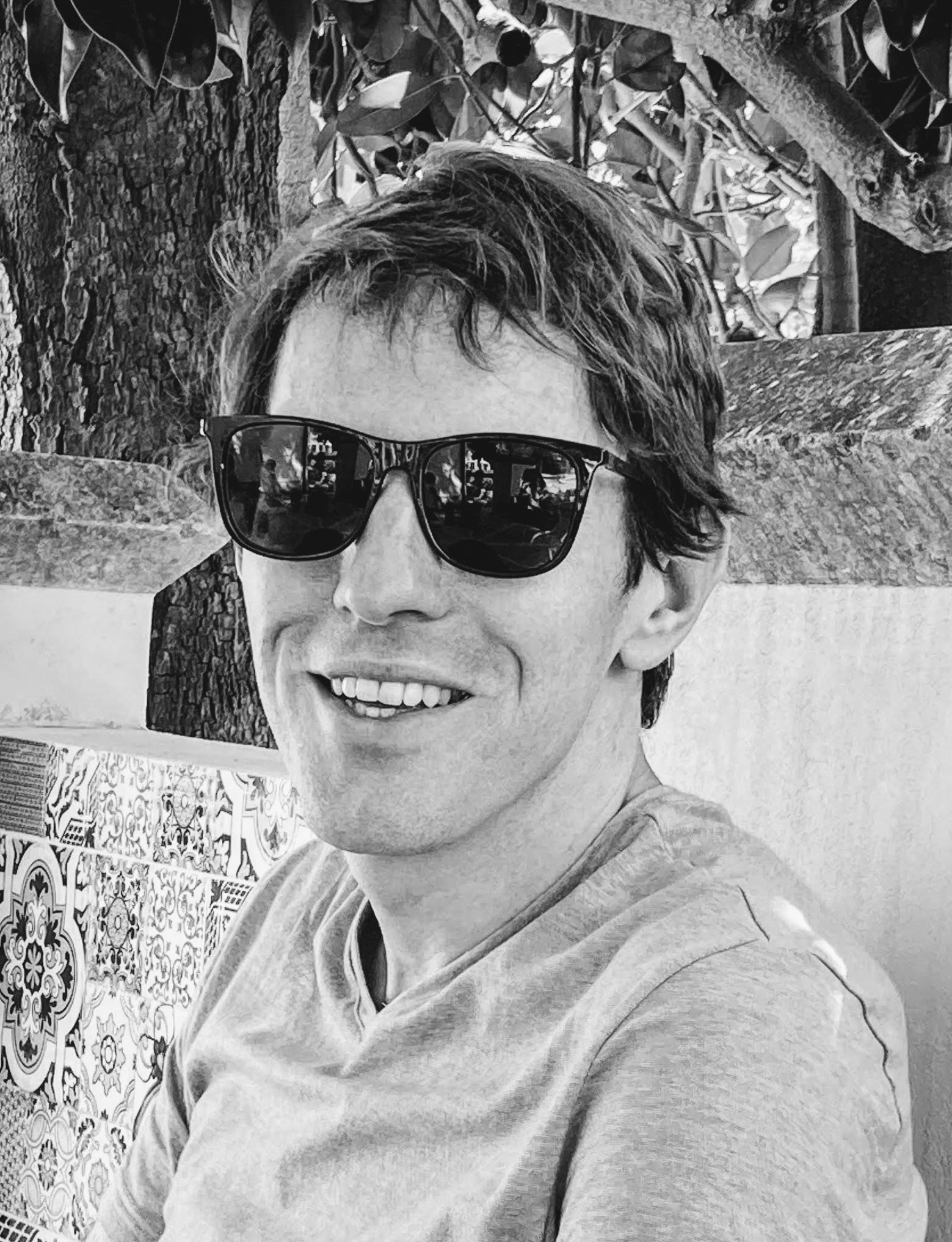
University of Oxford
Title: Combining Randomized and Observational Studies
Robins Evans is an Associate Professor in Statistics at the University of Oxford, and a fellow of Jesus College. He received his PhD in Statistics from the University of Washington in 2011, and he was a Postdoctoral Research Fellow at the Statistical Laboratory in Cambridge from 2011 to 2013. His research interests include graphical models, causal inference, latent variable models and algebraic, and semi-parametric statistics.
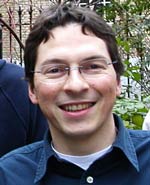
Institut Curie
Title: Reliable Causal Discovery from Information Theoretic Principles
Hervé Isambert is a research director at CNRS and group leader at Institut Curie, Paris. He received his PhD at Ecole de physique-chimie de Paris, and did a postdoc at Cornell and another at Rockefeller university. His on-going research concerns causal discovery and other data analysis methods for complex heterogeneous datasets with application to biological and biomedical data. His lab develops information-theoretic methods and machine learning tools (https://miic.curie.fr) to learn interpretable causal graphical models from large scale genomic data (single-cell multi-omic data), live-cell imaging data (tumor-on-chip experiments) as well as medical records of patients.
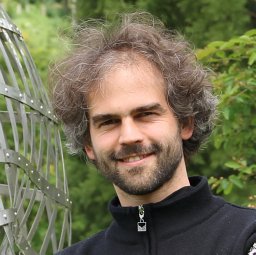
German Aerospace Center and TU Berlin
Title: Causal Inference for Complex Spatio-Temporal Systems
Jakob Runge heads the Causal Inference group at the German Aerospace Center’s Institute of Data Science in Jena since 2017 and is chair of Climate Informatics at TU Berlin since 2021. Jakob studied physics at Humboldt University Berlin and obtained his PhD at the Potsdam Institute for Climate Impact Research in 2014. For his studies he was funded by the German National Foundation (Studienstiftung) and his thesis was awarded the Carl-Ramsauer prize by the Berlin Physical Society. In 2020 he won an ERC Starting Grant with his interdisciplinary project CausalEarth. His research interests include causality and time series graphs, quantification of causal interaction strength, information theory, and application to climate data.
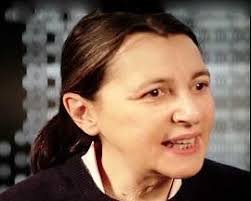
TAU, CNRS, Paris-Saclay University
Title: Causal Modeling through (i) Adversarial Learning and (ii) Inverse Covariance Matrix Decomposition
With a background in maths (Ecole Normale Supérieure), Michèle Sebag went to industry (Thalès) where she started to learn about computer science, project management, and artificial intelligence. She got interested in AI, became consulting engineer, and realized that machine learning was something to be. She was offered the opportunity to start research on machine learning for applications in numerical engineering at Laboratoire de Mécanique des Solides at Ecole Polytechnique. After her PhD at the crossroad of machine learning (LRI, Université Paris-Sud), data analysis (Ceremade, Université Paris-10 Dauphine) and numerical engineering (LMS, Ecole Polytechnique), she entered CNRS as research fellow (CR1) in 1991. In 2001, she took the lead of the Inference and ML group, now ML & Optimization, at LRI, Université Paris-Sud. In 2003 she founded together with Marc Schoenauer the TAO (ML & Optimization) INRIA project. Her research interests include causal modelling, preference learning, surrogate optimization, and machine learning applications to social sciences.
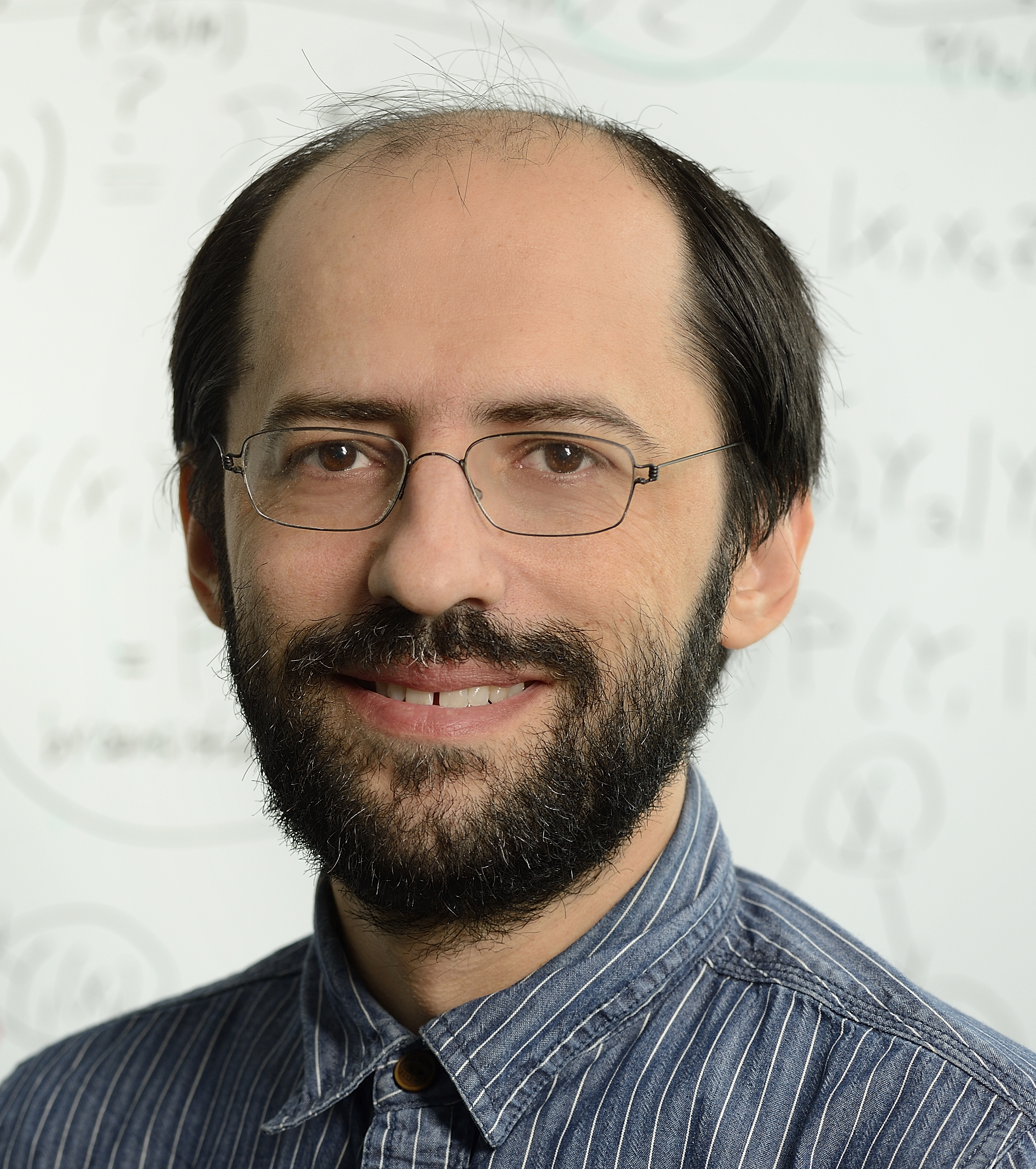
Johns Hopkins University
Title: The Proximal ID Algorithm
Ilya Shpitser is a John C. Malone Associate Professor in the Department of Computer Science in the Whiting School of Engineering at the Johns Hopkins University. He is a member of the Malone Center For Engineering in Healthcare. He graduated from UCLA where he had great privilege to be advised by Judea Pearl. As a postdoctoral scholar at the Harvard School of Public Health, he was fortunate to receive mentorship from James M. Robins.
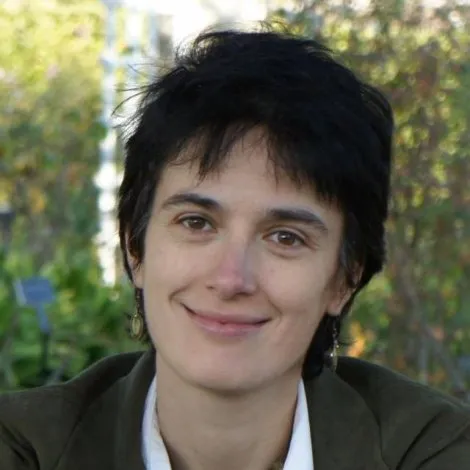
University of Cambridge, Alan Turing Institute, London
Title: Causal Deep Learning
Mihaela van der Schaar is the John Humphrey Plummer Professor of Machine Learning, Artificial Intelligence and Medicine at the University of Cambridge and a Fellow at The Alan Turing Institute in London. In addition to leading the van der Schaar Lab, Mihaela is founder and director of the Cambridge Centre for AI in Medicine (CCAIM). Mihaela was elected IEEE Fellow in 2009. She has received numerous awards, including the Oon Prize on Preventative Medicine from the University of Cambridge (2018), a National Science Foundation CAREER Award (2004), 3 IBM Faculty Awards, the IBM Exploratory Stream Analytics Innovation Award, the Philips Make a Difference Award and several best paper awards, including the IEEE Darlington Award. Mihaela is personally credited as inventor on 35 USA patents (the majority of which are listed here), many of which are still frequently cited and adopted in standards. She has made over 45 contributions to international standards for which she received 3 ISO Awards. In 2019, a Nesta report determined that Mihaela was the most-cited female AI researcher in the U.K.
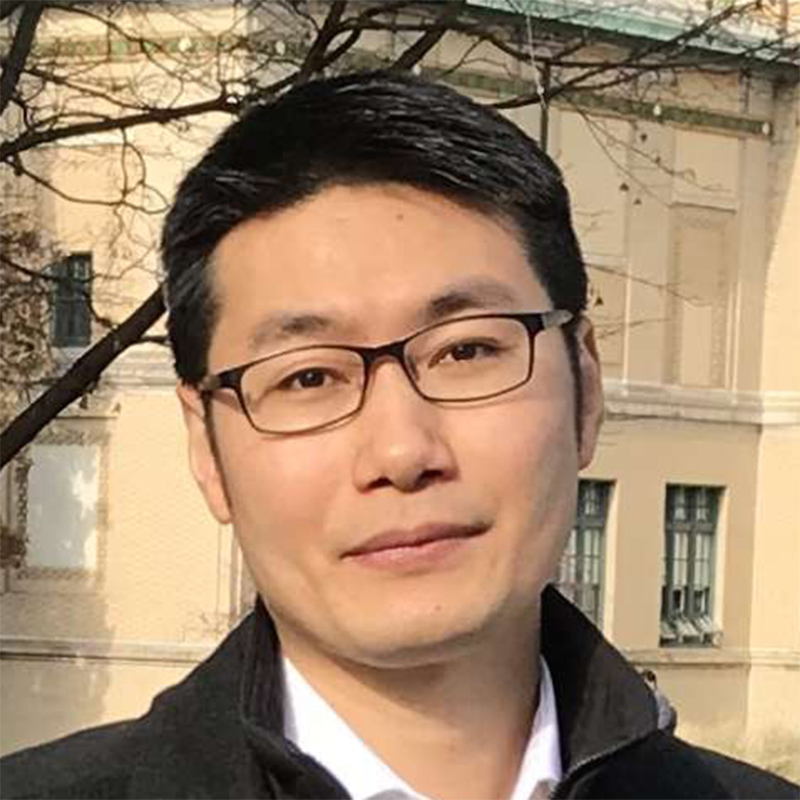
Carnegie Mellon University (CMU), Mohamed bin Zayed University of Artificial Intelligence (MBZUAI)
Title: Causal Representation Learning: Successes and Challenges
Kun Zhang is an associate professor of machine learning at Mohamed bin Zayed University of Artificial Intelligence (MBZUAI), on leave from Carnegie Mellon University. His research interests lie in machine learning and artificial intelligence, especially in causal discovery and causality-based learning. He develops methods for automated causal discovery from various kinds of data, investigate learning problems including transfer learning, concept learning, and deep learning from a causal view, and study philosophical foundations of causation and various machine learning tasks. Recently he has been focusing on causal representation learning. On the application side, he is interested in neuroscience, computer vision, computational finance, and climate analysis.

EasyVista

CNRS and University of Grenoble Alpes
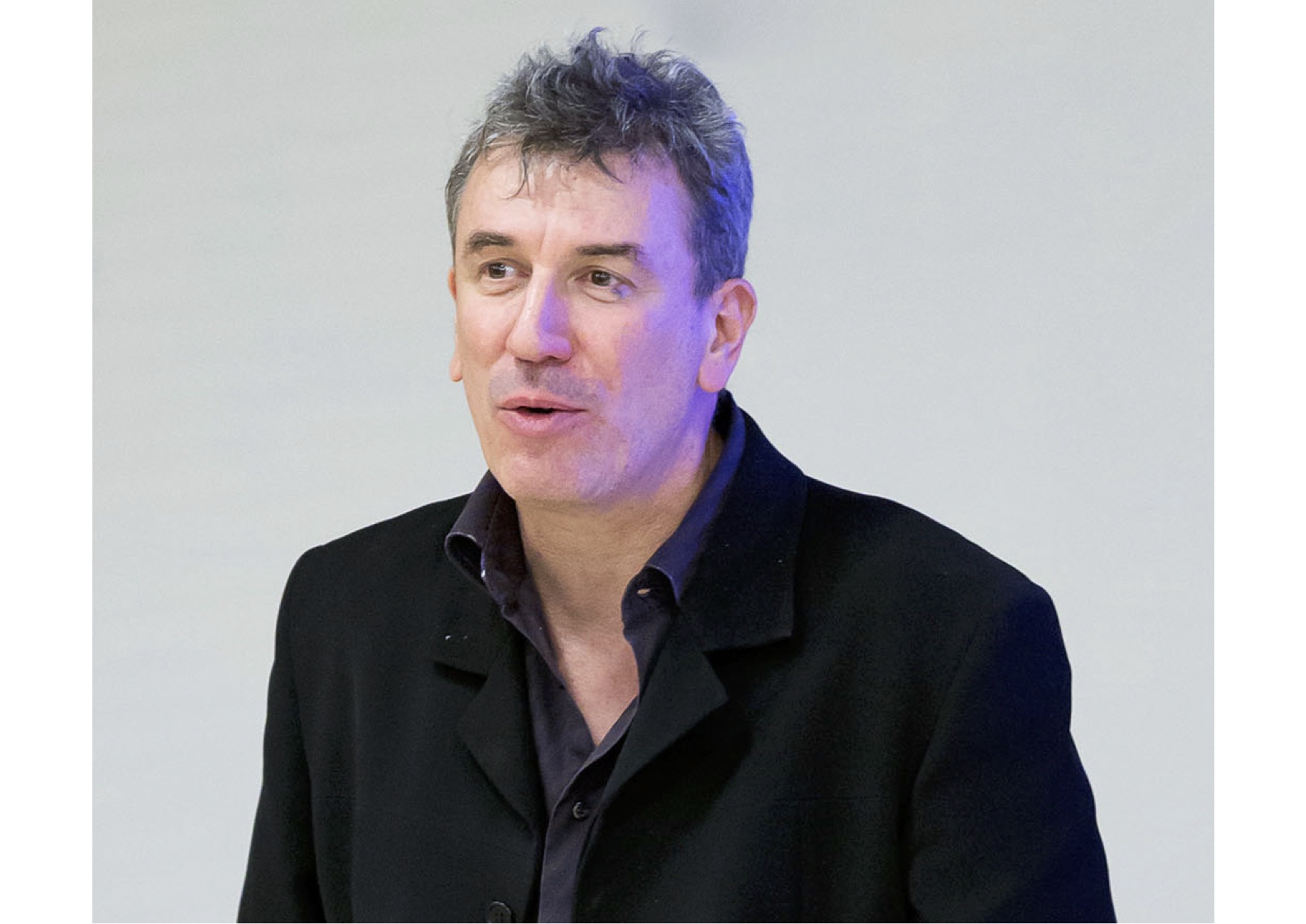
University of Grenoble Alpes

Inria
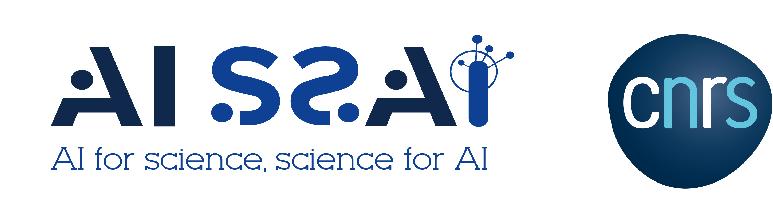
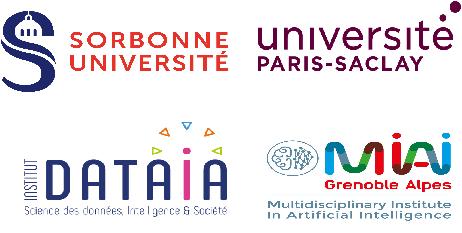
Code of Conduct
Our Fundamental Challenges in Causality is dedicated to providing a harassment-free experience for everyone, regardless of gender, gender identity and expression, age, sexual orientation, disability, physical appearance, body size, race, ethnicity, religion (or lack thereof), or technology choices. We do not tolerate harassment of participants in any form. Sexual language and imagery is not appropriate for any venue, including talks, workshops, parties, Twitter and other online media. Participants violating these rules may be sanctioned or expelled from the event at the discretion of the conference organizers. If you have any concerns about possible violation of the policies, please contact the organizers (organizers.quarter.causality@gmail.com) as soon as possible.